AI Needs More Women
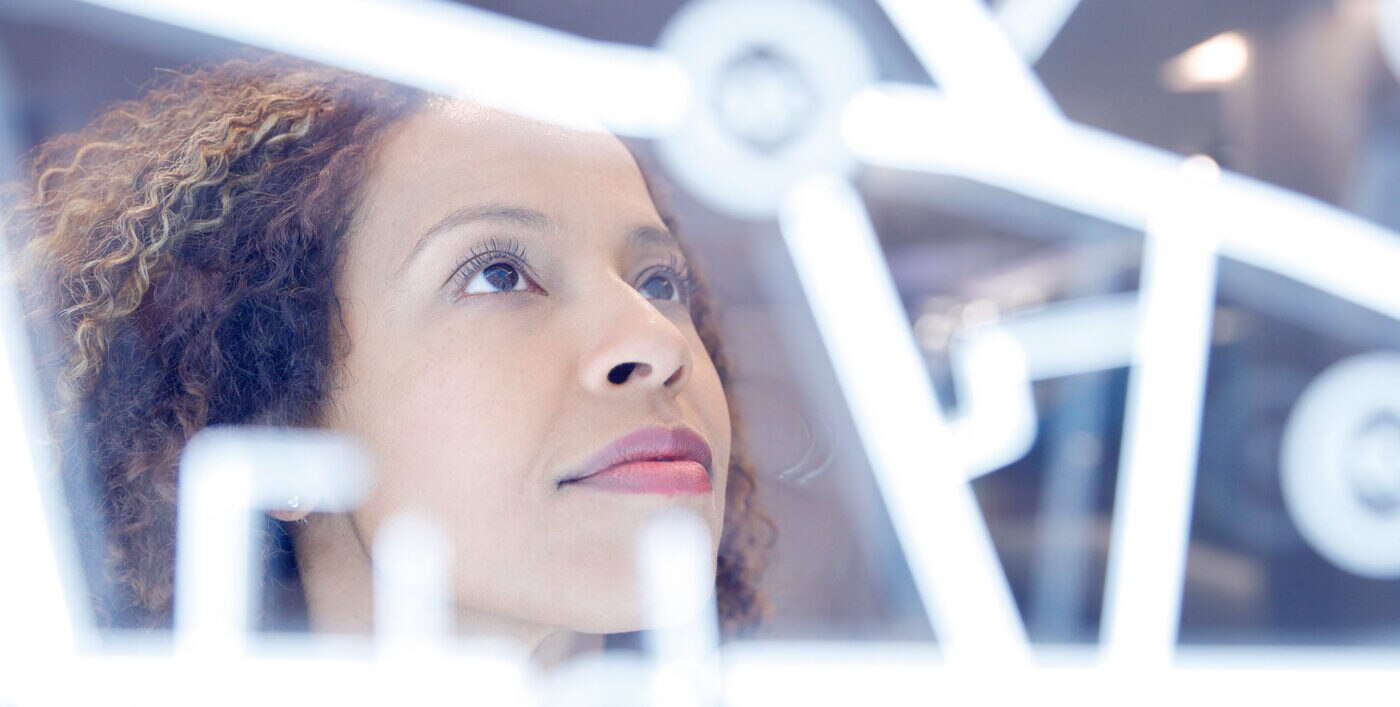
Spare a thought for those women currently struggling with ill-fitting personal protective equipment (PPE) on the front lines of the COVID-19 pandemic. A 2016 survey by the Women’s Engineering Society found that just 29 percent said the personal protective equipment (PPE) they wear at work was designed for women and 57 percent found that their PPE sometimes or significantly hampered their work.
Those statistics become less surprising when we’re told that most dust, hazard and eye masks are modelled on a ‘standard’ European and US male face shape. That’s just one particularly pertinent example of the gender data gap Caroline Criado Perez explores in her 2019 book Invisible Women.
Perez explores the impact a male-dominated and male-designed society has had (and continues to have) on data that is used to govern our everyday lives. Women, especially minority women, are frequently omitted from data still used today to train face and voice recognition systems, inform medical trials and—as with the examples above—influence the design of a product, drug, law or system.
Here’s another example: the automotive industry still relies on data from dummies modelled on the ‘average’ male physique and seating position, meaning that women are still 47 percent more likely to be seriously injured and 17 percent more likely to die than men in similar road traffic collisions.
Invisible women, biased AI
We could write these off as data problems. Any quantity of historical data great enough to be useful is likely to contain some form of conscious or unconscious bias that reflects the attitudes and priorities of those collecting that data—largely Caucasian males—at the time.
But it’s in the use of this data as training sets for machine learning (ML) that we’re experiencing a new problem: biased AI. When you ask a machine to learn from biased data, it will inherit that bias and apply it to the decisions it makes. In this blog, my colleague Noel Hurley explores how racial prejudice in historical court data led to racial bias in the US criminal justice system’s COMPAS ML algorithm.
There have been many cases of gender bias transferring from humans to AI applications. One key example is in word embedding, which is a popular framework for natural language processing (NLP) tasks used in voice assistants. A 2016 paper by Microsoft Research and Boston University found word embeddings trained on Google News articles exhibited gender bias to a disturbing extent, pairing words like ‘man’ with ‘doctor’ and ‘woman’ with ‘homemaker’.
This isn’t a data problem, it’s a human problem
Let’s not pretend, though, that the bias that originally affected this historical data has been eradicated from society. Prejudice is alive and well, as we’ve been reminded of all too well in recent weeks. This isn’t a data problem, it’s a human problem. And when it comes to AI, the majority of ML today uses supervised machine learning, with training data labelled by a human operator. In applying these labels, both conscious and unconscious bias can be introduced into a model resulting from even the most objective data set.
The problem is compounded further by evidence that ML algorithms don’t just reflect a bias, they amplify it. One study by the University of Washington fed an ML a deliberately biased data set containing images of people cooking. Images were 33 percent more likely to contain women. Once the algorithm had finished training, that disparity had increased significantly to 68 percent—suggesting that the algorithm was mistaking men for women purely because they were standing next to a stove.
Arm’s AI Trust Manifesto outlines a set of ethical design principles for ML and AI. It states that every effort should be made to eliminate discriminatory bias in designing and developing AI decision systems. Again, that’s not just a data problem, it’s a human one too.
Unbiased AI needs a diverse workforce
But it’s not enough for us to ask the AI industry, in which only 22 percent of AI professionals globally are female, to abide by these principles. AI aims to mimic human thought and rationale. If programmed by a non-diverse workforce, how can we ever hope to build machines that represent a cross-section of human society and don’t just reproduce or even amplify the conscious and unconscious bias of the most represented demographic?
Today is International Women in Engineering Day, and while it remains a celebration of the outstanding achievements by women engineers throughout the world, it’s also a reminder that if we are to overcome problems like AI bias, we need to encourage more women to work in STEM (science, technology, engineering and math) sectors.
We should all be frustrated to hear from STEM Women that the UK saw little to no change in the percentage of female engineering and technology graduates from 2015 to 2018. Only 15 percent of those graduating within those years were women. The same long-standing gender stereotypes that have led to biased data are steering girls and women away from science-related fields.
Arm has many talented female engineers. But we need more. We want to inspire future generations of women to pursue engineering and technology as a career. We recognize that a solid foundation in STEM is a proven path to upward mobility; yet it’s a path many young people, especially girls, don’t take. Together, working in partnership with organisations such as FIRST, Tech She Can and the Arm Schools Program, we can break down barriers, provide inspirational resources and connect underrepresented and underserved students, including girls to STEM role models.
I hope that in doing so, we take one step closer to ensuring that AI technology is developed by a workforce that is representative of every person affected by its application, now and increasingly in the future. And in turn, if AI is ever to be fully accepted and trusted by society, it needs to have been built by a workforce that is as diverse as possible. We need the input to be as inclusive and unbiased as the output.
Any re-use permitted for informational and non-commercial or personal use only.