What Are the Key Strategies for AI Vision to Tackle High Costs and Complex Data Processing?
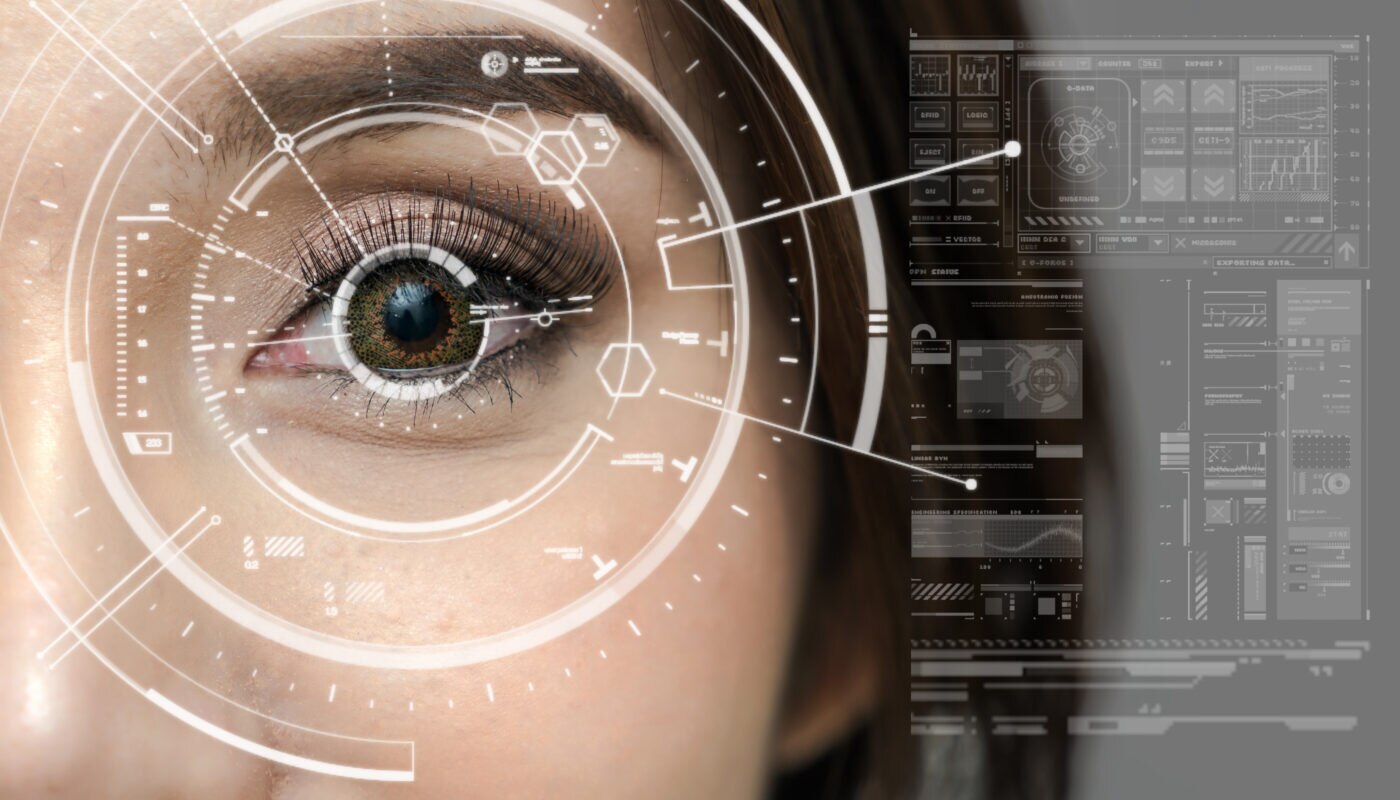
One of the most promising applications of IoT AI vision technology is to capture consumer data inside stores so retailers can more quickly and efficiently optimize product placement, store layout and customer experience based on video data.
But there are two major hurdles to overcome: Cost and complexity. A large grocery store that wants to harvest foot-traffic, purchase and other data would need about 15,000 cameras in store. At 30 frames per second of 4K video, those 15,000 cameras would produce 225 gigabits of data per second.
That happens because video data is enormous, compared with other forms of data, and intricate processing is required, including image recognition, object detection, and scene analysis. These AI vision tasks often require advanced algorithms and models, contributing to the computational complexity. On top of that, big data like that needs to be sent to the cloud for efficient computation and then back out for decision-making.
Clearly, 225 gigabits per second is uneconomical.
But that’s only if you think it’s still 2018, not 2023. Much has changed in the past five years. The combination of improved and more-efficient processing at the edge, coupled with AI and machine learning, now chips away at that big uneconomic roadblock in front of many promising vision applications.
How is AI Vision Transforming Edge Computing and Innovation?
Back then, too many vital technologies sat siloed, each either difficult or impossible to integrate with other important puzzle pieces to enable a frictionless innovation ecosystem. In a homogenous processing world it was difficult to be able to customize solutions for different vision workloads when one size had to fit all. What’s different about today?
Engineers and developers have attacked cost and complexity, as well as other challenges. Take the processing complexity challenge for example. One pathway to driving down the cost and complexity of vision solutions is to offer developers more flexibility in how they implement edge solutions – heterogeneous compute.
Designers are producing increasingly powerful processors that offer higher computational capacity while remaining energy efficient. These processors include CPUs, GPUs, ISPs and accelerators designed to handle complex tasks like AI and machine learning in sometimes resource-constrained environments. In addition, AI accelerators – either as a core on an SoC or as a stand-alone SoC – enable efficient execution of AI algorithms at the edge.
How is the Arm Mali-C55 Enhancing AI Vision and Edge Computing Synergy?
Let’s take one piece of the complexity puzzle. Arm in 2022 introduced the Arm Mali-C55, its smallest and most high-performance Image Signal Processor (ISP) to date. It features a blend of image quality, throughput, power efficiency, and silicon area, for applications like endpoint AI, smart home cameras, AR/VR, and smart displays. It achieves higher performance with throughput of up to 1.2Gpix/sec, making it a compelling choice for demanding visual processing tasks. And, when it comes to the push toward heterogeneous compute, the Mali-C55 is designed for seamless integration in SoC designs with Cortex-A or Cortex-M CPUs.
That’s key because in SoCs ISP output is often linked directly to a machine learning accelerator for further processing using neural networks or similar algorithms. This involves providing scaled-down images to the machine learning models for tasks like object detection and pose estimation.
This synergy, in turn, has given rise to ML-enabled cameras and the concept of “Software Defined Cameras.” And this allows OEMs and service providers to deploy cameras globally with evolving capabilities and revenue models tied to dynamic feature enhancements.
Think for example, of a car parking garage with cameras dangling above every slot, determining whether the slot is filled or not. That was great in 2018 for drivers entering the garage needing to know where the open slots are at a glance, but not an economical solution in 2023. How about leveraging the notion of edge AI and positioning only one or two cameras at the entrance and exit or on each floor and having AI algorithms figure out the rest? That’s 2023 thinking.
That brings us back to the retailer’s problem: 15,000 cameras producing 225 gigabits per second of data. You get the picture, right?
Amazon has recognized this problem and in the latest version of its Just Walk Out store technology, it increased the compute capability in the camera module itself. That’s moved the power of AI to the edge, where it can be more efficiently and quickly computed.
With this powerful, cost-effective vision opportunity, a grocery retailer might, for example, analyze video data from in-store cameras and determine that it needs to restock oranges around noon every day because most people buy them between 9-11 a.m. Upon further analysis, the retailer realizes that a lot of your customers – anonymized in the video data for privacy reasons – also buy peanuts during the same shopping trip. You use this video data to change your product placement.
How Will AI Vision Drive New Business Models and Optimize Edge Computing?
This kind of compute optimization – putting the right type of edge AI computing much closer to the sensors – reduces latency, can improve tighten security and reduce costs. It also can spark new business models.
One such business model is video surveillance-as-a-service (VSaaS). VSaaS is the provision of video recording, storage, remote management and cybersecurity in the mix of on-premises cameras and cloud-based video-management systems. The VSaaS market is expected to reach $132 billion by 2027, according to Transparency Market Research.
At a broader level, however, immense opportunity awaits because so many powerful potential applications have been waiting in the wings because of economics, processing limitations or sheer complexity.
Consider:
- Smart Cities: Video analytics for traffic management, pedestrian flow analysis, and parking space optimization in smart cities can lead to substantial data generation.
- Industrial Automation: Quality control, defect detection, and process optimization.
- Autonomous Vehicles: The sensors and cameras on autonomous vehicles, such as self-driving cars and drones capturing data for navigation and safety systems, perceiving their surroundings in real time.
- Virtual Reality (VR) and Augmented Reality (AR): Immersive VR and AR experiences require rendering and processing of high-resolution visual content in real time, resulting in significant data generation.
Leading-edge adopters aren’t waiting. In South Korea’s Pyeongtaek City, city leaders are planning to build a test bed using smart city technologies such as artificial intelligence and autonomous driving to be completed in 2025 and spread throughout the city.
The city of a half-million people grapples with traffic congestion and pedestrian fatalities. As part of a citywide “smart city” overhaul, experts have deployed Arm partner Nota.ai’s Nespresso platform – an automatic AI model compression solution – in vision devices to create an intelligent transportation system.
How is AI Vision Transforming Device Design and Consumer Experience?
At the device level, clever design is helping customers achieve their vision visions. Take the Himax Wiseye-II, a smart image sensing solution that can be deployed in a range of battery-operated consumer and home security applications, including notebooks, doorbell, door lock, surveillance camera and smart office. It marries Arm microcontroller and neural processor cores to drive machine vision AI more deeply into consumer and smart-home devices.
These examples and future innovation being designed today are happening because of amazing advances in edge AI technology. And in vision, they’re being built on Arm.
In addition to hardware, Arm makes the journey for developers of image solutions faster and more efficient, thanks for software libraries, interconnect standards, security frameworks, and development tools such as Arm Virtual Hardware, which allows them to run applications in a virtual on their target architecture before committing to hardware.
So remember those hopes of transforming the world with previously untapped amounts of data using vision technology that once seemed a far-off dream because of cost and complexity? They can become reality now.
Check out the possibilities for AI vision at the edge
Any re-use permitted for informational and non-commercial or personal use only.