Arm DevSummit: Tools, Standardization Key to Expanding ML
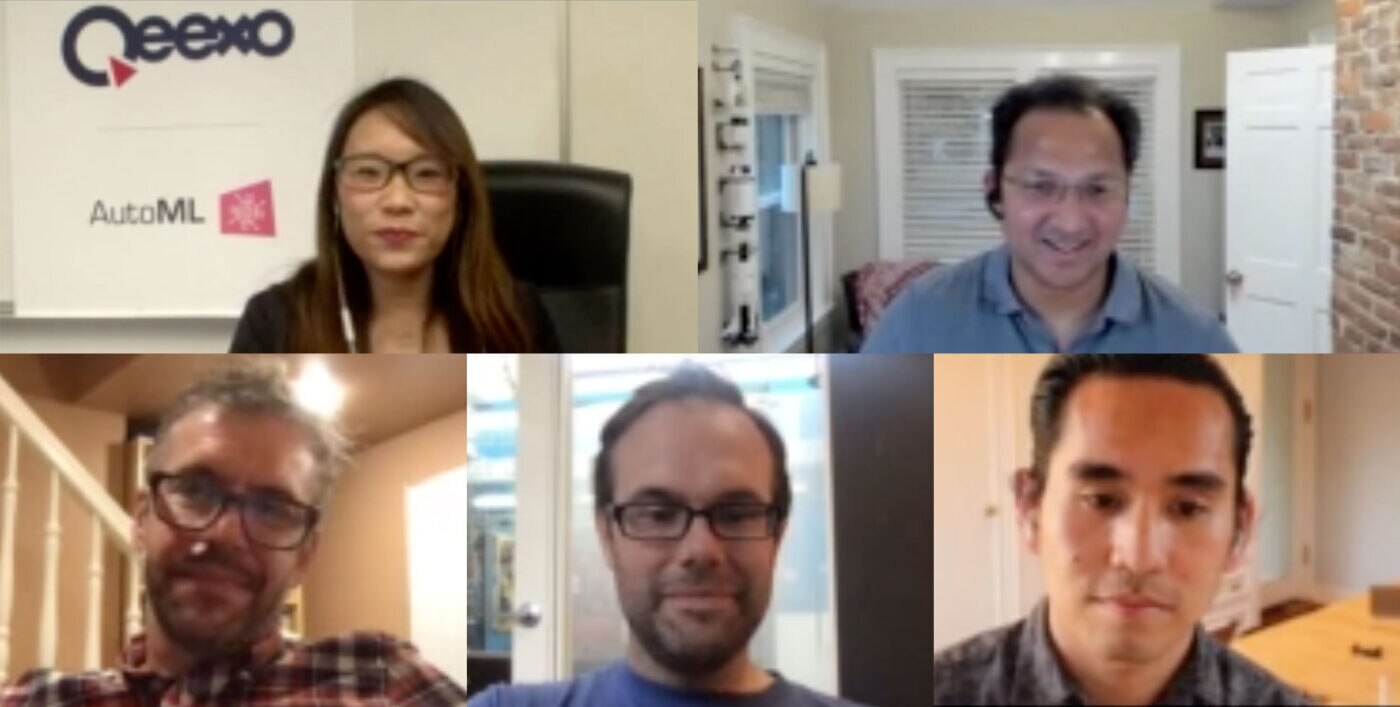
The opportunities to leverage machine learning at the edge and on endpoints is enormous but first, the industry must tackle tools, standardization and usability issues to minimize design challenges and put edge ML in the hands of more developers. That was the assessment of the Machine Learning at the Edge panel at Arm DevSummit 2020.
“Machine learning can become almost like a material for designing new experiences,” Gierad Laput, who leads the Interactive Sensing + ML research group at Apple. Right now, machine learning is in the hand of mostly engineers, but we can and should get it into the hands of everyone who dreams up new experiences so they develop the “vocabulary” and understand the limits of the ML “material,” he said.
“Once people start to understand that, I’m excited for a future where we get more magical experiences just because we’ve crossed the envelope of what means to use it as a tool, not as a hammer,” he said.
Chris Harrison, CTO and co-founder of Qeexo, a company whose technology aims to automate machine learning, said casting a wider net is key to scaling AI and ML and this can only be done by focusing on tools development.
“We desperately need better tools …. and automate the key tasks we do,” he said.
Why?
“Companies that make consumers devices – a KitchenAid, a toaster, a blender – they don’t have ML teams. They either need help from other companies or, better yet, the tools that can expose the incredible power of machine learning to their engineering teams,” he said. “Their teams are very capable, but they’re not ML engineers.”
It was a sentiment shared by all the panellists.
Jon Fry, senior director of technology strategy at Arm, said, “Empowering people to be able to deal with their ML edge problems requires a lot of technology underneath it,” including tools that help manage and deploy ML models, tools that enable different workstreams.
Four key constituencies
To truly move the market forward, we need to focus on four key constituencies, said Vijay Janapa Reddi, associate professor and visiting researcher with Harvard and Google:
- Apps developers, who need to understand the system their writing to.
- Device manufacturers, who need to understand what the microcontroller and software stacks look like and behave. This has to be done efficiently because “this ecosystem does not tolerate high cost,” he noted.
- Framework developers such as TensorFlow, who need to be freed from the manual tuning they need to do now on their runtime frameworks to take into consideration the heterogeneity of the hardware.
- IP companies such as Arm, who supply the elements for hardware but need to know the use cases to better serve partners.
“If you want to move all four groups forward … you need to have metrics,” Reddi said. “People need to agree on what is a standard benchmark, agree on what the machine learning task is, how you pre-processing, how do you do timing…the data sets you’re using.”
He noted that MLPerf, which develops benchmarks for measuring training and inference performance of ML hardware, software, and services, is one such industry group trying to make a difference in this area.
“It’s got to be a community-driven (standards and benchmarks) effort because this space is super heterogeneous,” he added.
Automation is key
“I do think the automation in order to create a cloud-native development experience is needed on (smaller endpoint) devices that don’t look and feel like a Raspberry Pi,” Fry said.
Harrison said the industry needs to automate as much as possible, and in fact, machine learning has automated processes in its own right – “really tedious data tasks,” as he put it. But right now, we’re not at a point where it’s fully automated; there’s a bit of “ML craftsmanship” that’s required today to optimize models and outcomes. “Humans in the loop lets you apply some ethics to machine learning…as well,” he added.
“We want to draw more people in; we want to expand the user baser. Machine learning is such a powerful thing we want to expose that to more and more people, and automation is going to make that easier,” Harrison said.
Any re-use permitted for informational and non-commercial or personal use only.