Demystifying the Power of Machine Learning in IoT
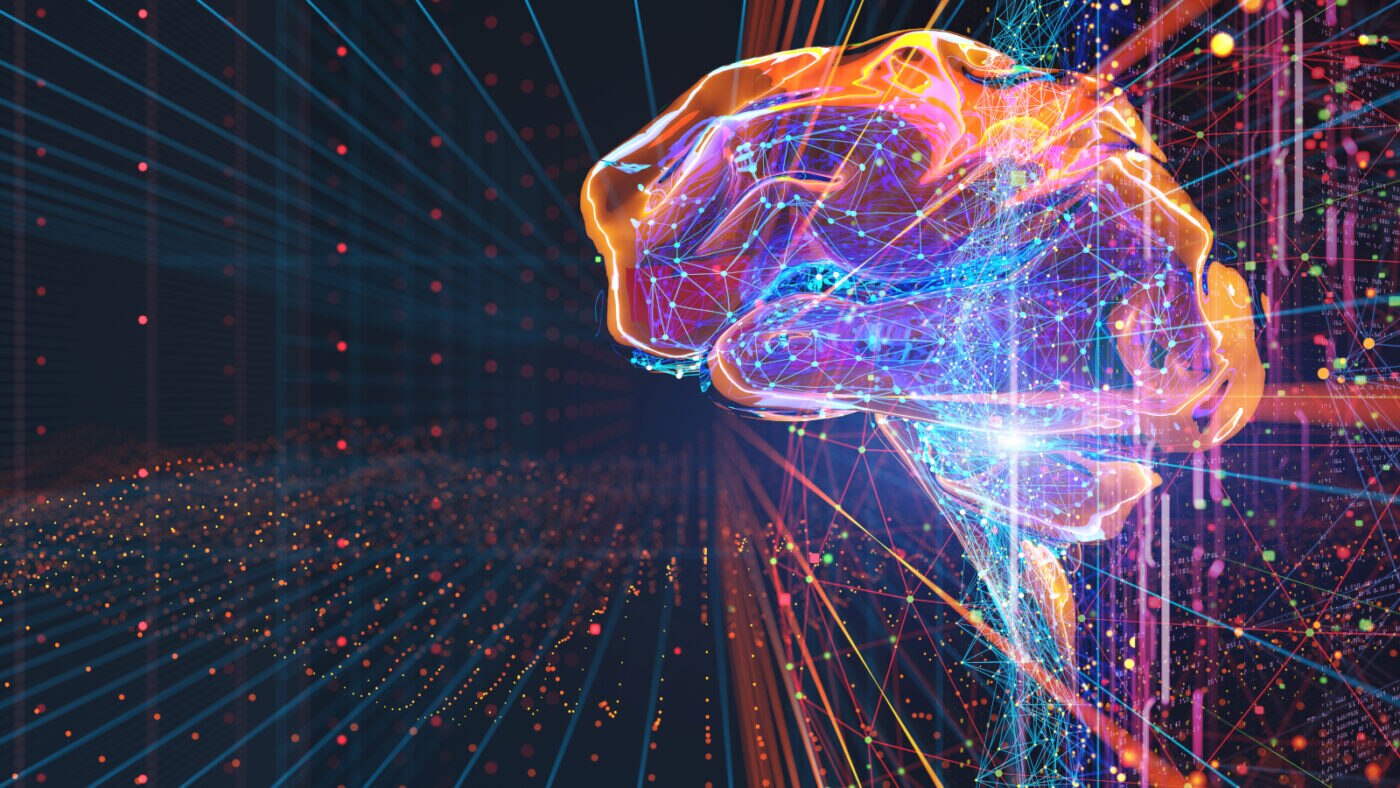
In recent years, the speed of innovation in the Internet of Things (IoT) has accelerated dramatically, paving the way of the integration of Machine Learning in IoT. The first major shift occurred when edge devices began to connect with each other. Now, these devices are connecting to the cloud, leading to a revolution in data processing. Vast volumes of data can now be processed more cost-effectively, while powerful algorithms are taking data interpretation to new levels.
This has resulted in creating systems in rainforests that can detect and locate illegal logging, identify the distress calls of endangered species, and even sense mechanical faults to alert plant managers before equipment fails – all in just the past few years.
How are Intelligent Systems Changing the Face of IoT?
We’re witnessing a powerful transformation across the IoT landscape. Devices are no longer solely dependent on cloud computing for data interpretation. Instead, they’re evolving into intelligent systems capable of making autonomous decisions based on the data they generate. This shift promises to expedite decision-making and enhance privacy and security by processing data closer to its source.
Take vision applications for example. A few years ago, home security cameras relied on cloud streaming to alert homeowners about package deliveries. Today, many modern home security cameras come with integrated machine-learning capabilities. This advancement reduces latency by eliminating the need to send data to the cloud and back. It also enhances privacy by storing personal information, such as images of a person’s home or belongings, securely on the device itself. Thanks to more powerful computing and machine learning on the device, the features of such systems are expanding. For instance, a camera would be programmed to capture images only when unfamiliar or new individuals are at the door.
Still not convinced about the rapid pace of innovation in IoT? Consider this: Some developers successfully ran a large language model (LLM) on a Raspberry Pi, one of the world’s most widely used IoT devices, and set up their own AI chatbot servers. To put it in perspective, an LLM, which consists of billions of parameters, is now operational on a Raspberry Pi. A step-by-step guide on this was published on Tom’s Hardware.
But this is just the tip of the iceberg. We will soon see various versions of language and transformer models being integrated into IoT edge devices with enhanced computing capabilities. This advancement will open doors to new possibilities that were previously thought to be purely imaginative. Let’s think of a few.
3 Ways Machine Learning is Transforming IoT
Strengthening security and privacy
As digital technology continues to advance rapidly and become an integral part of our lives, concerns about privacy and security have grown. It seems like every week we hear about a new hacking incident, whether it’s on a large scale like data centers, or a smaller scale such as IoT devices. The world took notice when reports surfaced of hackers accessing a baby monitor.
Many healthcare devices today monitor vital signs and provide health feedback. These applications must evolve with robust security measures to protect patient privacy. Thanks to advancements in machine learning, these devices will soon be able to analyze data directly on the device, identify patterns, and even customize based on individual preferences and goals. This could potentially lead to an increase in average life expectancy.
Improving local decision-making with faster response time
Local communities are witnessing substantial transformations with smart technologies. Smart cities use IoT sensors and devices to gather real-time data, aiding in informed decision-making. Machine learning algorithms analyze this data, offering real-time insights for effective decision-making. For instance, a smart transportation system can leverage ML to monitor traffic, detect accidents, provide alternative routes, predict traffic patterns, and suggest the best routes.
During the pandemic, the world turned to the convenience of online home delivery services. Meanwhile, smart robots and sensors were used across warehouses to identify packages for new orders and arrange optimal delivery. Soon, it is expected that ML-enabled IoT devices will address the last-mile delivery challenge, with drones delivering packages to remote households.
Optimizing operational efficiency
Even industrial factories, contributing to the global GDP, have become smarter over the years by adopting machine learning technologies to enhance operational efficiency in IoT devices. Predictive maintenance, a key aspect of industrial automation, uses ML to monitor equipment performance and predict potential issues, reducing downtime, boosting productivity, and cutting maintenance costs.
Smart home devices like Amazon Alexa and Google Nest have transformed home management, offering customized solutions based on individual needs. For instance, smart lighting systems can learn and adapt to user preferences. ML on IoT devices not only improves user experience but also helps reduce energy consumption by optimizing heat and lighting based on user behavior.
Challenges when integrating ML in IoT
However, some challenges need collective effort to overcome. One key issue is the use of multiple frameworks for model development, which often need to be compatible with various hardware devices. This can hinder scalability. To address this, Arm has been working on a new standardized operator called TOSA (Tensor Operator Set Architecture) so that devices can run future models developed on billions of devices. Arm also supports TVM (Tensor Virtual Machine), an open-source machine learning compiler framework that works on Arm’s CPUs and specialized ML accelerators.
Another challenge is data drift, which becomes more prevalent as IoT devices increase. Data drift happens when the data generated by IoT devices change over time due to factors including device wear and tear or environmental changes. This can alter the statistical properties or distribution of models and impact applications. However, with proper design, IoT devices can detect and classify these drifts, ensuring system reliability, performance, and safety.
Machine learning is revolutionizing the IoT, creating a vibrant ecosystem. Companies are inventing tools such as AutoML, specific models for IoT devices, and tools to translate these models across different frameworks, enabling them to run on IoT devices.
How is Arm Overcoming Scalability Issues in IoT?
Arm is collaborating with various partners to foster a thriving ecosystem for machine learning deployments in IoT devices. This includes partners for automatic machine learning, model providers, and end-to-end solution deployment, among others.
In the future, we expect all IoT devices to run some form of ML technology. That’s why Arm is enabling a broad range of ML devices. These range of tiny sensors running on Cortex-M CPUs to more complex systems using Cortex-A CPUs paired with custom ML accelerators, including GPUs, with even more devices on the horizon.
The integration of ML into IoT is transforming industries and our daily lives. This powerful combination is driving rapid growth and shaping a dynamic ecosystem where companies are developing AutoML tools, specialized for IoT devices, and frameworks for easy deployment. Another key aspect is the monitoring of the vast number of IoT devices running ML algorithms. This allows for the detection of data drifts, which can then be used to retain and redeploy models, further improving their accuracy and performance.
Together, ML and IoT are poised to reshape the world and unlock unprecedented possibilities across various sectors. To learn about the opportunities in this evolving age of innovation, click here for more information.
Any re-use permitted for informational and non-commercial or personal use only.