Unlock the Future of AI with Heterogeneous Computing
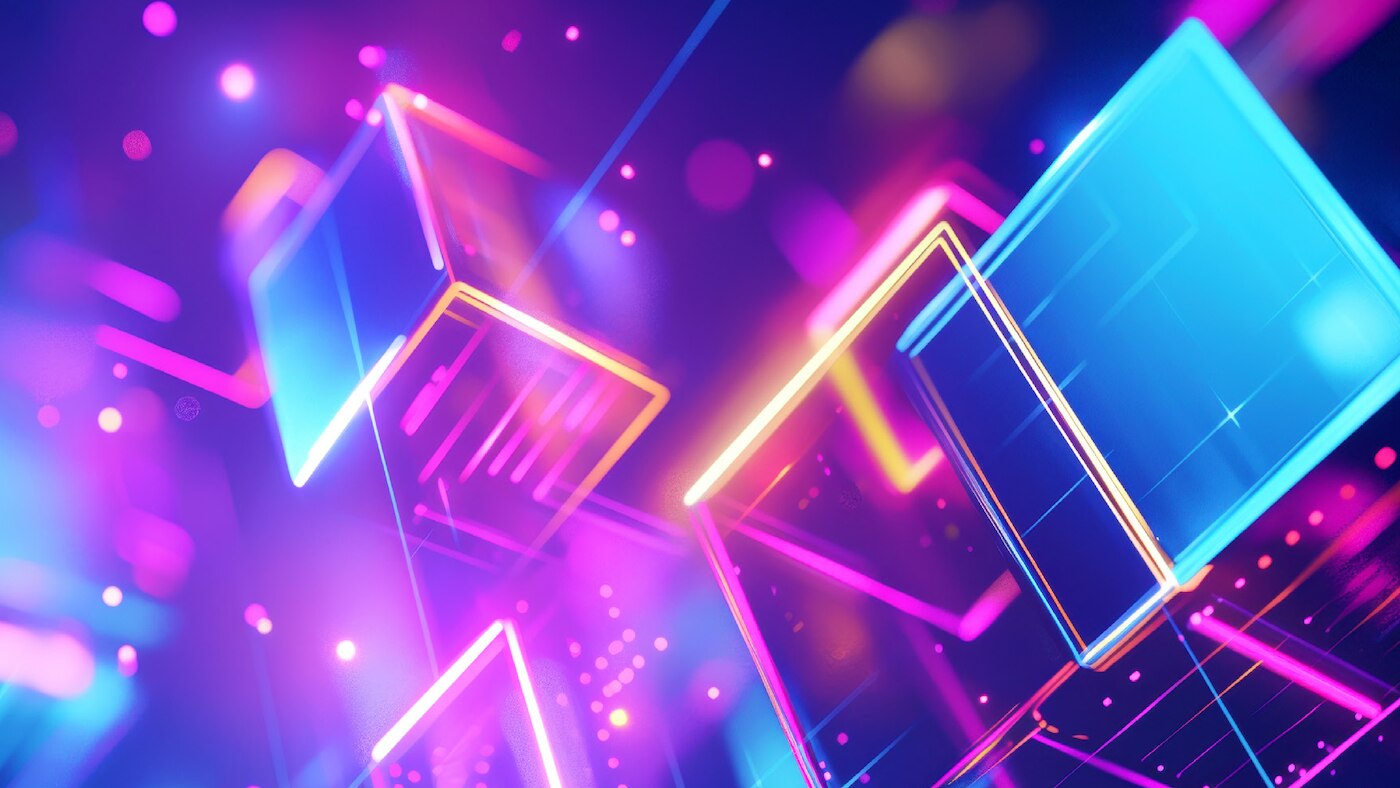
Artificial Intelligence is no longer just a research topic — it’s a daily reality. From personalized healthcare and smart wearables to immersive digital entertainment and autonomous robotics, AI is reshaping how we live, work, and create. But as AI applications grow more sophisticated, the underlying infrastructure must evolve too.
That’s the focus of the new MIT Technology Review Insights report, The Future of AI Processing, produced in partnership with Arm. The report offers timely insights into how businesses are rethinking their compute strategies to keep pace with the demands of AI today — and prepare for what comes next.
Why heterogeneous computing?
At the heart of this shift is heterogeneous computing — an approach that distributes AI workloads across different types of processors, such as CPUs, GPUs, NPUs, and other AI accelerators. Each component brings unique strengths: CPUs handle orchestration and general-purpose tasks or energy-efficient inference, GPUs power through training and high-volume operations at scale, and NPUs are optimized for real-time inference.
This architectural mix enables compute systems to dynamically match workloads with the most suitable processor, optimizing for performance, power efficiency, and cost.
As Ian Bratt, Vice President of Machine Learning Technology at Arm, notes in the report: “Heterogenous computing is for performance and efficiency. You might have a workload you’re running on one component that it’s well suited for, and one part might be better suited for a different component”.
AI at work, at play, and everywhere in between
The report highlights how heterogeneous computing enables smarter, more efficient AI across a variety of applications. For example:
- Wearables and smart home devices use compact on-device processors for real-time inference, while offloading heavier tasks like personalization and pattern recognition to the cloud.
- Industrial robotics in agriculture and manufacturing rely on a mix of computer vision and machine learning, with heterogeneous compute delivering low latency and optimized energy use in dynamic environments.
- Entertainment platforms such as streaming and gaming distribute inference, encoding, and personalization across CPUs, GPUs, and cloud infrastructure to balance performance and cost.
- Applications like voice assistants, predictive text, and real-time translation benefit from hybrid AI processing, which shifts from centralized cloud to edge and on-device compute. This approach improves response times, enhances privacy, and boosts energy efficiency.
This distributed model, powered by heterogeneous compute, allows AI to scale efficiently and adapt to real-world demands. Striking the right balance is crucial as AI models grow in size and complexity. In many cases, processing inference in the cloud is ideal — especially when using large models, handling high volumes of data from multiple sources, or needing to roll out updates quickly across a broad user base.
Built for a smarter, more efficient future
As highlighted in the report, power consumption and cost-efficiency are becoming critical concerns. With datacenter energy use projected to rise significantly, businesses are looking to do more with less. Heterogeneous compute enables them to manage workloads intelligently — reducing the need for brute-force GPU scaling and unlocking savings that can be reinvested into innovation.
This flexible approach also supports long-term adaptability. As workloads evolve, organizations need platforms that won’t lock them into fixed paths or force expensive overhauls. Heterogeneous computing architectures offer the versatility needed to adapt without compromise.
Explore the full report
The MIT Technology Review Insights report provides a deep dive into this new paradigm, with perspectives from leaders at Arm, Meta, AWS, and Samsung. If you’re planning infrastructure for the next generation of AI-powered products, this is a must-read.
MIT Technology Review Report
Explore business strategies to meet today’s AI workload demands.
Any re-use permitted for informational and non-commercial or personal use only.