Wildlabs: Building a Better World with ‘Bad’ Data
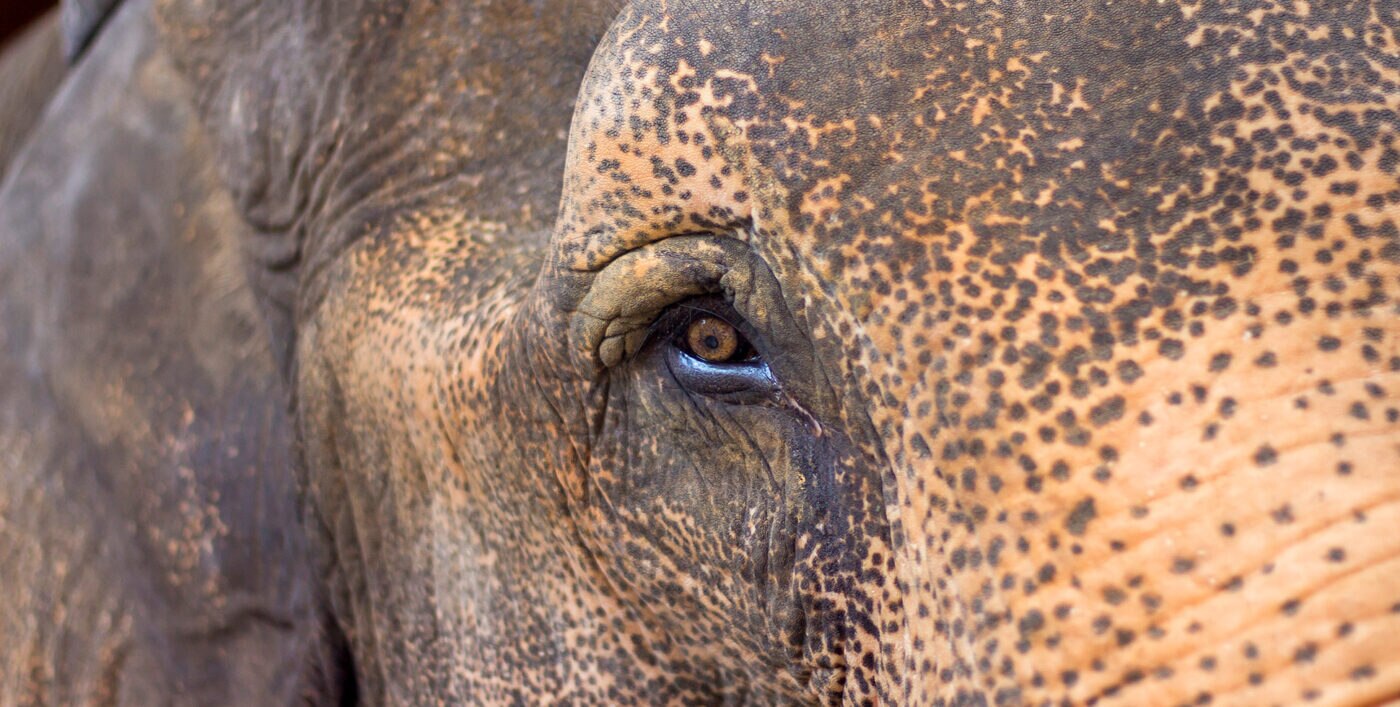
If your smartphone camera could only capture images 80 by 60 pixels in resolution, you’d be clamoring an upgrade.
But when it comes to helping establish peaceful coexistence between humans and wildlife, that minimalist resolution is right on target, says Alastair Davies of the Arribada Initiative.
Arribada has been developing a rugged, low-powered IoT system to provide villagers with an early warning system for potentially dangerous wildlife. In Ittoqqortoormiit, Greenland, the system is being tested to detect polar bears. In Asia, the target is Asian elephants—which can trample crops or injure people.
An 80 x 60 passive thermal sensor can correctly identify elephants 80 to 90 percent of the time from a distance of 20 to 30 meters, along with an image clear enough for human identification and verification.
“The low data rate of these pixel images in a resolution I can work on in a microcontroller means I can shrink models down in size and use thermal technology to detect the presence of an animal, use machine learning to teach the algorithm what the animal is and then generate a confident alert and transmit that to the community,” said Arribada’s Alastair Davies during a presentation at Arm DevSummit 2020 (available to registered attendees until November 23rd, 2020).
Hardware for a Harsh World
Conservation may be one of the most important applications for technology but it’s also one of the most difficult to serve. Internet of things (IoT) systems like Arribada’s need to be reliable enough to function 24/7, link to a whatever communications happen to be at hand, run on a trickle of battery power and recharge themselves via solar if available.
They also have to be able to withstand extreme conditions—operating at -50 Celsius in Greenland or being able to survive tropical storms in India—while producing meaningful results with a minimum of hardware resources. Minimizing false positives, like mistaking a cow for a predator, is also critical.
An 8 x 8 pixel array served up images that were pixelated, indistinct and blurry, Davies noted. Something warm has entered the frame, but it’s difficult to tell exactly what. Scaled up to 80 x 60, the distinct profiles of each species becomes clear while minimizing compute resources. (For the record, Arribada collected over 36,000 photos over a four-month period at a zoo habitat and field-tested it in Assam, India. 112 hours were also spent labelling data.)
The same tension can be seen in the design of FieldKit from Conservify. Born out of a National Geographic project in Botswana, FieldKit is an open-source, modular data logger specifically designed for the exacting and unpredictable world conservation research.
The core of the platform is an Arm Cortex M4-based board that will accommodate sensors for water levels, radiation, weather, soil moisture, air quality, temperature or other parameters along with a bevvy of peripherals, storage options and networking protocols. Scientists can assemble, deploy and synchronize FieldKit stations in around three hours.
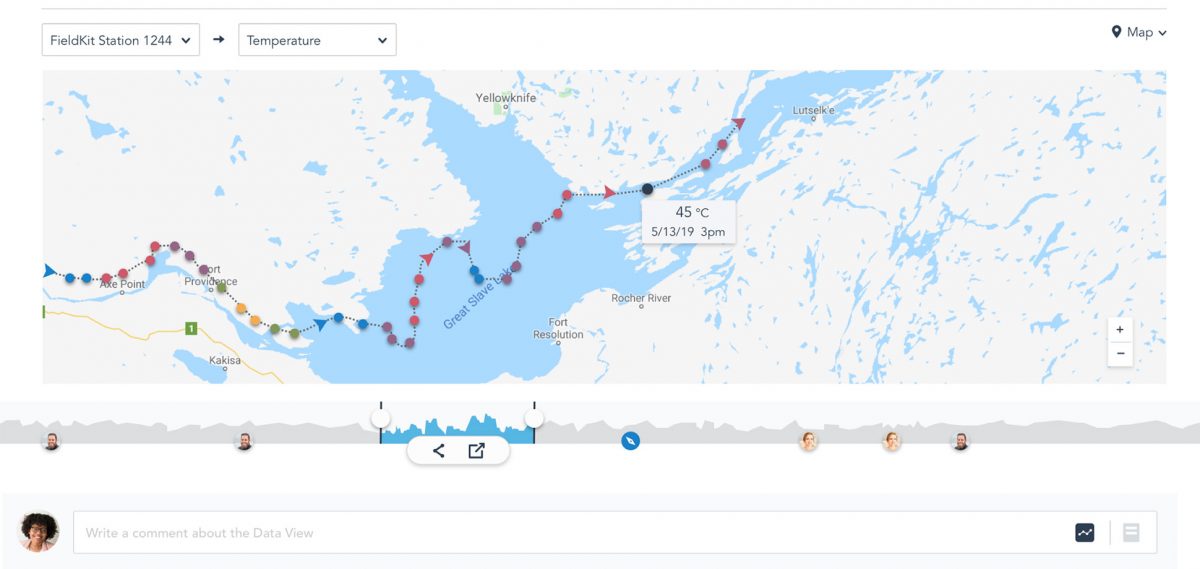
“Modularity in scientific sensors has never been easy to do,” said Shah Selbe of Conservify. Historically, scientific sensors have been reliable, proprietary and expensive, or flexible but not scalable.
“(Your device) has to function perfectly in a desert or a rainforest,” he added. “It’s a space that’s ripe for innovation.”
The hardware platform is complemented by an app that helps users set up their systems. The app also attaches metadata to data gathered by researchers with a FieldKit station so others can probe the information and possibly use it in their own experiments. In essence, the app creates a framework for making raw data more reliable. (You can order a FieldKit station soon.)
A website featuring data visualizations prepared by Jer Thorpe, who has served as a data artists for the New York Times and Smithsonian, is coming soon.
AI in the Wild
Even a few years ago, running effective artificial intelligence (AI) or machine learning (ML) algorithms on small devices was impractical. Researchers, however, have been both increasing the power/performance of their hardware through higher-performance CPUs and NPUs while reducing the code footprint of machine learning models. In some experiments, researchers have been able to achieve a 10x reduction in the size of models with only a marginal loss of accuracy.
In the next phase, Arribada will continue to fine-tune its technology as well as hand it over to WildLabs, the software and developer community for environmental research. (Arribada is also running a project to record data on the pollination process of Andean orchids, which is under threat of climate change and could impact other species.)
Conservify, meanwhile, is encouraging people to think of new sensors or modules for expanding the platform.
“You could have a California edition of FieldKit that’s monitoring fire activity or seismic activity,” said Shah. “the state of conversation in the world is not going as well as we hoped…We need all the help we can get.
Watch Wildlabs’ session at Arm DevSummit 2020
If you’re registered for Arm DevSummit, you can still watch the Wildlabs session, Conservation Technology, until November 23rd.
Any re-use permitted for informational and non-commercial or personal use only.