Cambridge Consultants: How We’re Pushing the Endpoint AI Envelope
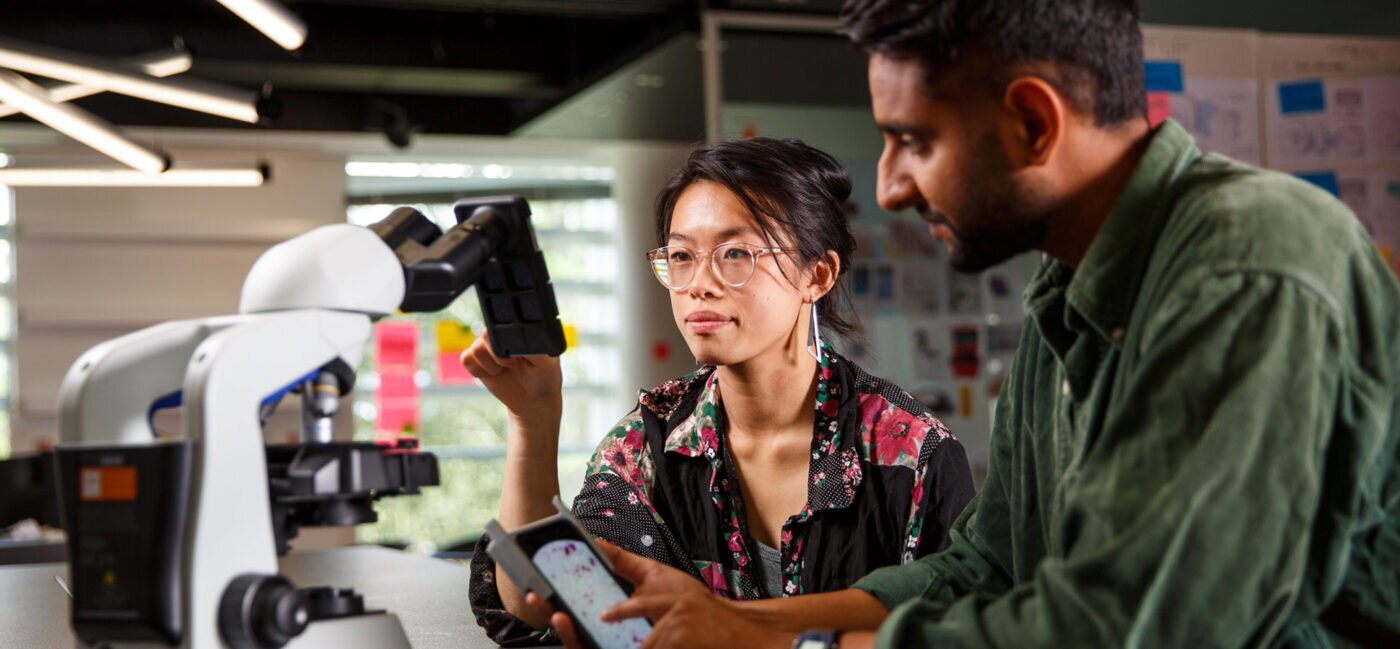
Artificial intelligence (AI) may have grown up in the cloud but delivering transformational products and services means taking AI out of the data center and into the real world.
As one of the world’s leading product development and technology consultancy firms, our technologies can be found in homes and hospitals, in satellite networks and even inside the human body. Many of these applications now use endpoint AI, enabling us to turn raw sensor data into context and meaning on the device itself without sending it to the cloud.
The results of a year of socially-distanced, determined experimentation with the Cortex-M55 and Ethos-U55 aren’t just impressive—they’re game-changing.
But enabling this level of intelligence in endpoint devices with stringent size, cost, power and connectivity constraints is no small task. It requires two key things: robust silicon and a deep understanding of the design trade-offs in power and performance to maximize the latter while maintaining or even reducing the former.
When Arm announced the Cortex-M55 processor and Arm Ethos-U55 micro neural processing unit (NPU) exactly one year ago today, we jumped at the chance to see just how far we could push the power-performance envelope. The results of a year of socially-distanced, determined experimentation with the Cortex-M55 and Ethos-U55 aren’t just impressive—they’re game-changing.
Cortex-M55 + Ethos-U55: A step-change in what’s possible with endpoint AI
As an Arm Approved Design Partner, it wasn’t long after the launch last February that we were able to put this new AI duo through its paces.
Our initial research involved migrating our ultra-low power Voice Activity Detection (VAD) reference design from the Cortex-M3 to the Cortex-M55 and Ethos-U55. We wanted to draw comparison with earlier platforms that we were familiar with to explore the capabilities.
We quickly achieved a remarkable 7 times reduction in average power, yet 1,000 times increase in core speed. It was clear to us then that this wasn’t just the next generation of Cortex-M microcontroller: this was a step-change in what’s possible in endpoint AI.
On-device voice detection that doesn’t need to send all its data to the cloud has major benefits in latency, privacy and power, and this kind of voice detection is going to become increasingly important to the consumer market in the coming years.
The incredible uplift in performance we experienced in porting our VAD reference design to the Cortex-M55 and Ethos-U55 opened up a number of new previously impossible avenues, such as including vision alongside voice detection.
But it also gave us the confidence to really see how far we could stretch the capabilities of these chips.
Pushing the limits of AI medical applications at the endpoint
Putting scepticism firmly to one side, we began to wonder if we could port something as large and complex as a cloud-based deep learning application to this microprocessor duo, and in doing so prove that with the right optimization and silicon IP, even complex neural networks can be deployed on very low power edge devices.
The application we chose centered on a concept system developed by Cambridge Consultants to improve treatment monitoring of tuberculosis (TB) in resource-limited countries by combining AI with a smartphone to capture images from a laboratory microscope. Stained sputum sample images were originally analyzed using a deep learning algorithm in the cloud to identify, count and classify infected cells to determine the disease state of the patient.
To give you an idea of scale, this treatment monitoring application is 350 times more computationally complex than a typical object detection application using the MobileNet V2 neural network, which is commonly used in industry. MobileNet V2 requires a single inference per image of around 0.8 billion multiplier-accumulators (MACs), whereas this research required 70 inferences of around 4 billion MACs each per image.
The port was not only successful: we achieved similar run times and accuracy levels to the application’s former cloud deployment yet drew just a few Watts in the process. These power reductions were achieved through understanding and optimizing the network implementation during the translation and quantization stages, which had a dramatic effect on the run-time, power consumption and accuracy during the cloud to endpoint migration.
Wide-ranging applications for endpoint AI
The applications for this research are huge: real-time medical AI can be deployed in low power endpoint devices and used in settings where Internet connectivity is unavailable, or bulky and power-hungry computing equipment would be impractical.
It also opens the door to combine further benefits of processing AI data on endpoints, including lower latency and lower power. All whilst leaving room to improve privacy and security, since data does not leave the users device.
This research is directly comparable to many other applications and markets, enabling device manufacturers to move complex AI workloads into everyday consumer devices, factories, and even smart cities. This is a topic discussed further in Cambridge Consultants’ recent whitepaper.
From signal processing in billions of mobile phones, to AI in smart inhalers, Cambridge Consultants has generated billions of dollars of value for our clients, by creating and optimizing world-leading silicon platforms. As an Approved Design Partner and Functional Safety Partner, we consider it our duty to see how far we can push the latest Arm IP in order to demonstrate to Arm, our customers and the world just how powerful endpoint AI can be.
Unlock the Benefits of Artificial Intelligence for IoT Devices
Arm offers new compute technologies coupled with software and tools to help companies streamline the design, development, and support for AI-based IoT applications.
Any re-use permitted for informational and non-commercial or personal use only.