How to Automate End-to-End ML for Edge Devices
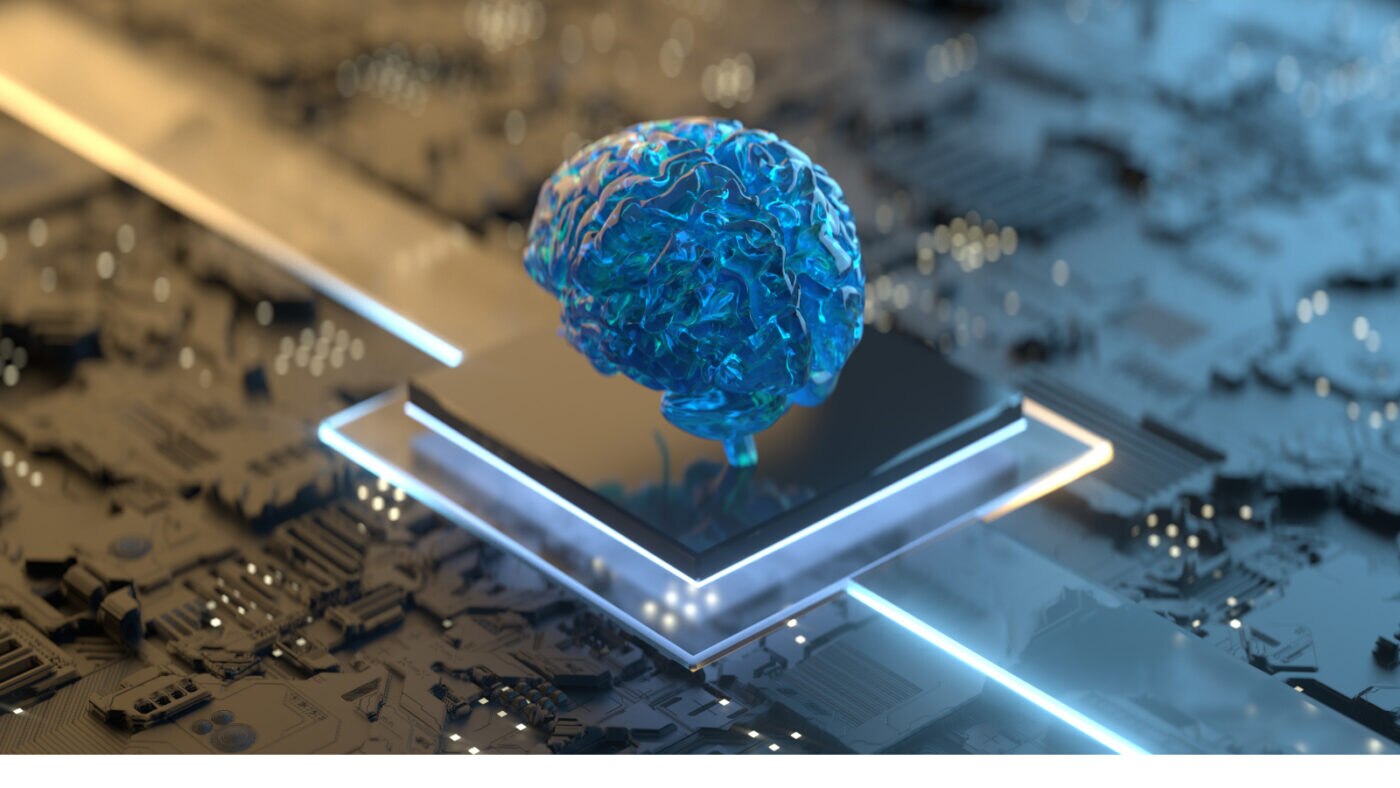
Hardware fragmentation loomed as an early barrier for developers who wanted to quickly scale their edge AI ambitions.
TDK Qeexo, which develops machine learning (ML) solutions for applications including industrial, manufacturing, embedded systems, and mobile devices, learned this challenge first-hand. The company started in 2012 with its first product, FingerSense, a mobile phone technology that could discriminate between different types of touch input using vibration sensing and capacitive touch.
“We created this excellent machine learning application, and our customer said, ‘Great! Listen, we need you to support something like 60 handset models per year,’” said Michael Gamble, Director, Product Management, TDK Qeexo. “And we said, ‘hold on. This took us something like four months with a team of ML experts to support this one handset.’ But at Qeexo, we don’t take challenges lying down.”
To address these challenges, TDK Qeexo developed Qeexo Auto ML, a fully automated, end-to-end machine learning development platform leveraging sensor data to enable the rapid build and deployment of lightweight machine learning solutions – tinyML – running locally on embedded devices. For edge AI, it is a no-code environment that enables data collection, visualization, annotation, model training and deployment and a whole host of other useful features.
By automating the most tedious parts of machine learning development process, they were able to reduce the time and effort required to support multiple handset models. This automation also allowed them to scale their business to support over 400 million handsets.
Streamlining edge AI development
With Qeexo Auto ML, teams without machine learning support can easily add it to their repertoire. Enterprises benefit from faster iteration cycles and reduced costs by eliminating the need to hire expensive embedded machine learning resources. The automated platform enables subject matter experts through machine learning professionals to perform tasks traditionally done by specialized resources, providing a significant cost advantage.
Qeexo, which was purchased by TDK in early 2023, has collaborated with Procter & Gamble (P&G) to deploy Qeexo AutoML for P&G teams to create intelligent solutions for consumer products. P&G presented at the spring 2023 Tiny ML Summit, highlighting how Qeexo’s software reduced the activation energy required to add intelligence to their products.
One unnamed customer was so impressed by a demo video created by TDK Qeexo’s CEO – showcasing its capabilities in discriminating between different motions – that it engaged with the company. This led to the development of a condition-based monitoring solution for that company’s industrial machines.
In the spirit of knocking down development barriers, TDK Qeexo has a close collaboration with Arm for hardware IP and development support. They have integrated Arm Virtual Hardware – a family of functionally accurate representations of Arm-based processors, systems, and third-party hardware– into their Qeexo Auto ML platform. This allows users to prototype with just software, without the need for physical hardware.
“At the moment, Qeexo AutoML powers specific hardware devices,” Gamble said. “So with the Arm Virtual Hardware, we’re aiming to overcome these boundaries and create a manufacturer agnostic, virtual, configurable sensor module from Qeexo AutoML.”
2023 Edge AI Technology Report
Edge AI, empowered by the recent advancements in artificial intelligence, is driving significant shifts in today’s technology landscape. This comprehensive report lays out the challenges and opportunities.
Any re-use permitted for informational and non-commercial or personal use only.