Bearly Scratching the Surface: AI’s Role in Wildlife Conservation
Summary
In the first of a two-part series, Brian Fuller hosts wildlife researcher Melanie Clapham and Arm’s Ed Miller to discuss the intersection of AI technology and wildlife conservation, particularly focusing on the BearID project, which the pair co-founded. They explore how machine learning is being used in wildlife conservation to identify individual bears through camera traps, the challenges of data collection in the field, and the ethical considerations of working with First Nations communities. The discussion highlights the importance of collaboration and the potential of AI to enhance wildlife research and conservation efforts.
Speakers
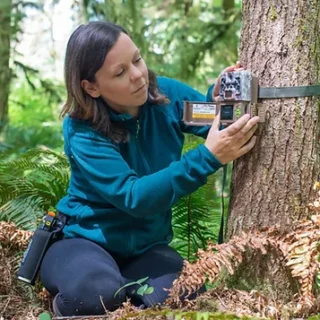
Melanie Clapham
Melanie Clapham is a conservation biologist with the Nanwakolas Council specializing in applied large carnivore conservation using non-invasive research techniques. Her current research is focused on developing advanced monitoring techniques for bears (the BearID Project). Through her research, she has the opportunity to work alongside First Nations on wildlife-monitoring programs and facilitate the inclusion of scientific knowledge in management decisions. She also works closely with the ecotourism industry in British Columbia to assess and reduce the impacts of commercial viewing on wildlife.
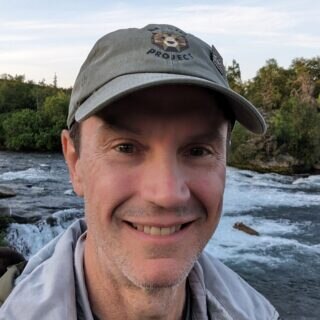
Ed Miller
Ed is a Senior Principal Engineer at Arm and a volunteer developer for the BearID Project. As part of the Strategic Alliances Technical Marketing team at Arm, he utilizes his background in both hardware and software to manage technical relationships with key partners. For the BearID Project, he develops AI applications to accelerate research in conservation science. He is passionate about the environment, wildlife and photography. Ed holds a BS degree in Computer Engineering from Carnegie Mellon University.
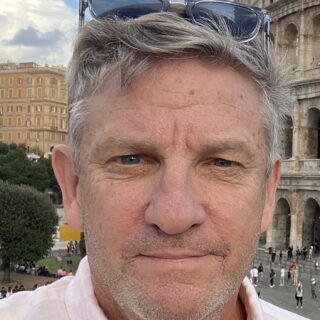
Brian Fuller, host
Brian Fuller is an experienced writer, journalist and communications/content marketing strategist specializing in both traditional publishing and emerging digital technologies. He has held various leadership roles, currently as Editor-in-Chief at Arm and formerly at Cadence Design Systems, Inc. Prior to his content-marketing work inside corporations, he was a wire-service reporter and business editor before joining EE Times and spending nearly 20 years there in various roles, including editor-in-chief and publisher. He holds a B.A. in English from UCLA.
Transcript
Brian: Hello and welcome to the Arm Viewpoints Podcast, where we explore technology topics at the intersection of AI and human imagination. I’m your host, Brian Fuller, Editor in Chief at Arm. In the first of a two-part series, we’re diving into facial recognition, not of humans, but of bears, and how technology is revolutionizing conservation efforts around the world.
My guests are Melanie Clapham, a conservation biologist and postdoctoral research fellow at the University of Victoria, Canada, and Ed Miller, a senior principal engineer at Arm and co-director of the BearID project, which he co-founded with Melanie. In this fascinating episode, we’ll talk about the origins of the BearID project.
And now it combines wildlife biology with cutting edge AI technology, the challenges and benefits of using camera traps to study bear behavior and populations, the magic of bear cam chat group. How AI and machine learning models are being adapted to identify individual bears, the role of hardware, including armed technology, in enabling AI powered wildlife monitoring, ethical considerations when working with indigenous and other communities on conservation projects, the impact of this technology on global bear conservation efforts, and much, much more.
I can barely wait, and I promise you that’s the only pun you’re going to hear from me. So, without further delay, we bring you Melanie Clapton and Ed Miller. So, Melanie, Ed, welcome. Thanks for spending some time with us today.
Melanie: Absolutely. Happy to be here.
Brian: Before we, we dive into this very meaty subject, um, tell us a little bit about yourselves, your background.
My background. Um, and how you got where you are now, Melody.
Melanie: Yeah, I’m, um, Melanie Clapham. I’m a wildlife biologist for the Nanwakolas Council, which is a collective of six Indigenous First Nations on the west coast of what is now British Columbia, Canada. Um, I’m also a co-director and conservation scientist for the BearID Project.
Um, and I came over from the UK to Canada about 15 years ago to study grizzly bears. And I’ve been doing that ever since. And most of my research focuses on bear behavior. So, I look at things like communication of bears, interactions with people. Um, I’m more recently looking at things like their habitat use and their movement.
Brian: Why bears? Why not gorillas or something like that?
Melanie: Good question. Coming from the UK, we don’t really have any large carnivores left. Um, so definitely always been drawn towards, uh, larger mammals. I guess for me, it’s with bears. I feel like the more time you spend studying them and the more time you spend around them, the more interested you become.
So, more and more questions you just want to be able to answer and address. They’re really fascinating animals, they’re really charismatic animals, they’re often quite misunderstood animals as well. So, I feel like by studying them, gathering more information and understanding about, around them. Um, we can really, really help, um, change people’s attitudes towards them and therefore their conservation.
Brian: And they’re so cuddly looking.
Melanie: They’re quite fuzzy. Yes. People like their ears, especially people always say, yeah, they’re, they’re really beautiful animals as well. Absolutely.
Brian: So, my colleague Ed, I know, uh, primarily from a lot of the technology writing that he does online, but he’s also an active participant in our annual Movember competition inside the company, and I’m not looking forward to competing with him again.
This year, Ed, tell us a little bit about yourself.
Ed: Thanks Brian. Um, Ed Miller. I’m a senior principal engineer here at arm. Uh, and I also volunteer and I’m co director and a developer at the BearID project, which we co-founded with Melanie. Um, I started my career developing hardware, um, many years ago at this point.
And, uh, over the years migrated to a lot more software and management and eventually more of kind of external technology partner relationship management roles, which is my current role at Arm. I have a degree in computer engineering from Carnegie Mellon University, and I am definitely very passionate about wildlife.
The environment and pretty much anything that is trying to make the world a better place for the humans and the animals that live in it, including fighting men’s health problems with Movember
Brian: and we live vicariously through your awesome wildlife photos. So, thanks for that. Um, You’re obviously not strangers to each other.
You co-founded the BearID organization. Tell us about how that came to be and why.
Ed: From my standpoint, I started my journey in machine learning probably around 2016. You know, it was kind of the hot topic of the time, and I wanted to come up to speed with it. And as part of my learning process, I wanted to work on a project that I could develop from scratch, because that’s the only way you really know what you’re doing.
Around the same time, I got hooked on a bear cam. So, the Brooks Falls brown bear cam from explore. org. It was something that I would get on, you know, to scratch my wildlife itch when I would have a few spare moments. And so, I would watch this cam, and it’s located in Katmai National Park in Alaska. And it really captured my attention for a lot of the same reasons Melanie described earlier about why bears and they are just so fascinating.
And that bear cam has a chat group, and the chat group would talk about, you know, which bear is on the camera and, you know, initially I’m just thinking, these are just, they’re bears. I can’t tell the difference. What, what are you talking about? And eventually I would start to learn to recognize their behaviors and recognize some of their symptoms.
specific physical traits and got to a point where I could identify these bears myself. And that was kind of my aha moment of, well, if I can do this, then a computer must be able to do this as well. So, in typical fashion, I scraped the internet to find some bear pictures from these cat, my bears, which, which had, you know, individual labels to them.
I tried to build a machine learning model using human face detection methods and, uh, had some, you know, mild success with it. It was looking very promising. And so, I thought, okay, what do I need for machine learning? I need more data. So, I joined this wildlife community called wild labs and I started asking around about labeled data of bears.
And lo and behold, I think around the same time, Melanie had joined the same platform, and we were put together by the platform community manager, their staff. And, um, yeah, from my perspective, the rest is sort of history. What was your journey there, Melanie?
Melanie: Yeah. So, uh, from my perspective, I mentioned earlier that I’ve done quite a lot of work with that behavior, um, using image-based data as well.
So, we, we use camera traps, um, All the way back, back in 2009 when I first came over to, to Canada to study them, uh, we were putting camera traps on places that bears come to communicate. So, specific trees that they’ll come and they’ll mark their scent against, and then other bears will come and investigate that scent, and they use that to communicate.
So, part of that work involved identifying individual bears. Which I’m sure we’ll talk more about in terms of how complex it is, and it can be and how much time we have to spend actually studying images of individuals. And from my point of view, actually being out in the field and trying to see these individuals so that we can recognize them when we see them on cameras.
So, it’s a really kind of complex process and a lot that we have to do just to be able to say this is one specific bear that’s we’re seeing on camera and showing this behavior and this is another one. So, kind of over time working on this project, I began to think, well, if I can start to recognize, I guess it’s really fun to just hear Ed’s explanation because it was a similar kind of concept for me is if I’m able to start to recognize these individual bears by seeing images of them by seeing them in person.
over time and seeing how they change in different seasons and year to year. Um, I wonder if there’s an automated way of doing this and, you know, I’m from a behavioral, a behavioral, a biology and ecology background, so I didn’t have a clue about any of the technology side of things. And, you know, when we first started thinking about this, um, and I started to look into this, you know, concepts of, uh, biometrics for animals.
I was really only able to find papers on things like facial recognition for people, and it had just been started to be looked at for, um, primates, so for chimpanzees and gorillas as well. So, it was really kind of in its infancy back then of applying these techniques, um, to wildlife, and so I just wondered if it might be possible if we can do it for some primate species, could we do it for bears as well?
Um, but I didn’t have a clue, how do I go about that? I’m, you know, I don’t have those skills myself. So, again, as Ed said, that’s why I joined the Wild Labs Network, which was kind of just becoming to be established then, to try and meet some like-minded folks to find out, you know, is this possible? And as Ed said, then we, we became introduced and we, got talking amongst ourselves and realized our objectives were really closely aligned.
And we thought, well, we should pull our resources together and create the BearID project. The rest is history.
Ed: One interesting side note on that as well. This, this actually happened even before I joined Arm, but as it turns out, uh, Arm is one of the major sponsors of Wild Labs, which is a group within the Flora and Fauna Foundation in the UK.
And so that was a very interesting thing when I, when I found out early on that, uh, my new employer was, was a sponsor for this site that I, that I find is, adds such value to the conservation and technology community.
Brian: Your hiring was meant to be some stars. Yeah, this, this whole topic is fascinating to me because about a year ago, might’ve been two years ago, we did a story on a company called Gravity and they were prototyping a similar animal identification.
AI based camera, and the story behind that was the CEO had just moved to Armenia and one of the first weekends there, he took his family to a wildlife refuge. Where they have 10 snow leopards. And I think there are only a thousand snow leopards in the world left. And he started talking up the ranger and the ranger said, yeah, we, we, we follow their migratory patterns and their reproductive patterns.
We have cameras out in the woods. And. The executive sort of perked up at this and then the ranger said, well, it’s a real pain in the neck because we have to go out. They’re not really digital. I mean, they are digital to a certain degree, but we get a lot of false positives because they capture everything that sets off the sensor.
If we could have a camera that just identified snow leopards, wouldn’t that be great? Well, the guy goes back Monday, and he gathers a team together and says, hey, we’re going to make a prototype. Let’s pivot from, from your work to the AI for Bears challenge and Fruitpunch AI. Let’s, let’s bring in that part of the story.
Tell us about how that came about.
Ed: Yeah. So, uh, you know, up until now, BearID is, has been mostly running on. servers and workstations. Um, and you know, Melanie does most of the, uh, the grunt work of going out into the field and getting SD cards from the cameras and loading them onto PCs and, you know, of course, doing all the work of identifying and pulling out which species are on there and finding the bears and identifying the individuals.
And so, we’re, we’re alleviating some of that with the BearID project for, for the individual ID. But the ultimate goal would be, okay, how can we bring this to a more real time situation where the, you know, on the camera itself, we can run some of these, some of these models and do some of this identification.
It was through, uh, through the Fruit Punch AI who run AI challenges where they build basically a program to develop a product, like a 10 week cohort, which they bring in, uh, do some education and then bring a bunch of new learners to bear on a on a project like this, and we thought this was a good way for us to start experimenting with different methods on how we could get some of these models into a suitable hardware device that could run in the field.
And so, we, we did a We, we worked with Fruit Punch AI to set this up. We created a data set for them to be able to use for, for training. And we gave them some specific parameters around, okay, we want to have this type of model capability running on these types of devices.
Brian: Easy as pie. And Fruit Punch AI is doing a number of these challenges is my
understanding. Is that correct?
Ed: Yes, they have a lot of different what they would say AI for good challenges. Many of them are wildlife related, but some of them are, you know, reducing poverty or improving water conditions, or I think identifying, uh, Wildfires or, or, or oil spills, all kinds of different projects that they run things on and they coordinate them with groups that are doing the work out in the real world and have the data sets necessary.
And then bringing in these new learners that are trying to build their careers in, in artificial intelligence applications and giving them real world projects to work on that have some, you know, some good in the end.
Brian: So, the fun part about doing these podcasts is you get to do a bunch of research. And so, I wanted to research camera traps and they’re not new, or at least the mechanical versions of them aren’t new.
There was a guy in the United States named George Shiras III, not the first, not the second, but the third, who in the, in the 19th century created a tripwire-based camera, and he would catch wildlife. Photos using nighttime flash photography and at the time National Geographic was mostly a text-based publication he sent in his photos, and they were over the moon about them and so they published a bunch of Photos of his in the early 1900s.
He eventually became a fellow of National Geographic and is considered one of the fathers of wildlife photography. Flash forward to now, where we’re putting these battery-powered digital computers, basically, uh, out in the wilderness doing great work. Let’s talk a little bit about the work that they’re doing.
They’re obviously capturing, um, images and you’re, you’re using that data, more data, the better, um, talk about how the identification works. Cause I think of a bear, and I think, Oh, it’s a bear. I’m going to run. Don’t run. That’s
Melanie: the first thing you don’t do from a bear is run.
Brian: Unless you’re with somebody else who’s slower than you.
Is that the old joke?
Melanie: Sorry, that I hear that run and bears and I know straight away.
Brian: Okay. Duly noted. Do not run. So, so you’re identifying the bears. Does it get as, uh, obviously you can do different types of bears. Can it do gender differentiation? Talk a little bit about this.
Melanie: Yeah. So, um, I mean, at the moment, our main species that we’re working on is grizzly bears.
So, brown bears. Um, we have a couple of different data sets for brown bears, um, from a few different places. So, we’re trying to gather as many images from different regions where the bears, where brown bears live. Uh, because they can look slightly different in different regions as well. So, we have some data from North America, some from Europe as well.
So, yeah, so your concept is, you know, you put your camera trap out in the fields. Uh, we place our cameras in a certain way so that we capture, we try to capture a nice image of a bear’s face as it’s walking past, um, and these camera traps have sensors. So, when something that’s warmer than the external environment moves past, it kind of trips some of the beams.
Um, and starts to record. Um, and we use videos and as you can set cameras to take photos or to take videos. Um, we use like 20 second short video clips so that by the time that bear has moved past the camera, we’ve got hopefully, um, a nice image or a few nice images of the face, uh, within that, and then we can go and collect the memory cards from those cameras, uh, which we do manually at the moment.
We haven’t really, we don’t use at the moment, like embedded cameras. Um, but we have to actually go out and. Collect the memory cards, bring those back, and then go through the footage, as I’ve mentioned earlier, and begin to process who’s who in the images. Um, and, and that’s what we use as our kind of training and test data.
Brian: And what are you, what are you looking for from that data once you’re, once you synthesize it all?
Melanie: Yeah. So, I mean, it completely is dependent on what specific research questions you have. And that’s one of the great things about camera traps is that they can be used to address a real wealth of different ecological and management questions about wildlife.
So, specifically for the BearID project, we’re interested in being able to detect specific individuals on camera and be able to track their movements of those individuals. across different camera sites so that we can examine movements of bears across landscapes and begin to ask questions about things like their habitat use, um, how their movement changes in different times of year, and also to try and identify important areas for bears.
Um, because brown bears aren’t territorial, so they don’t just stick to one specific area, but they have home ranges and their home ranges overlap. So, in certain areas that have really good food availability, we can see quite a few different individual bears using one site. So, it can give us an idea of how important certain areas, um, on the landscape are for bears.
Um, also another benefit is if you can recognize individual bears on camera traps, you can do population estimates as well. So, that’s something at the moment that has been missing for bears because in using camera traps to study populations. So, it’s been used for quite a while for animals that have patterns on their bodies.
So, spots and stripes that way you can individually identify them quite easily, relatively easily. Um, but for what we call unmarked species, like brown bears and other bear species that don’t have these distinguishing body patterns or body marks, it’s been very difficult to recognize them. Therefore, we’ve had to rely on other techniques like DNA, DNA sampling to be able to conduct population estimates.
So, again, if we’re able to use camera traps and I confidently identify individuals. We can do population estimates, we can start to study their movements, so it kind of opens the door for addressing lots of different ecological questions about them.
Brian: From a high level, I’m thinking long term, will these technologies be now out in the field almost in perpetuity so that you can capture years’ worth of data, or is it not at that point yet?
Melanie: Yeah, hopefully. I mean, that’s what we have going on our study site. I mean, we’re quite lucky in terms of bears hibernating over the winter. So, we have a bit of a down period where we take most of the cameras down and we’re able to spend some time focusing on the data. Um, for other species that don’t hibernate, obviously you just leave your cameras up, um, all year round and just incrementally bring in this data, start to process it, and our approach is, you know, we go through the data, we manually process it initially, and then we’ve got.
Um, data then that we can use for training and testing the models until eventually we’re ready for the models just to take over that, um, all that workload.
Brian: What, what sort of learnings have you, have you gotten from the data or is it, is it too early yet?
Melanie: Um, well, we’ve actually just started to look at, um, like I say, kind of a bit of a proof of concept of tracking individual bears, um, across kind of space and time, so we’re using a core dataset.
Um, from one of the regions where I study bears, um, on the West coast of BC. And so, we’ve got kind of a core set of around 50 different individuals. And so, we’re starting to be able to kind of track their movements, as I say, now on cameras. Um, we can do it at small kind of watershed levels. And then we’re now testing how we can kind of scale that up across different watersheds.
So, initially trying to track these specific bears that we know, and that we can recognize. And then eventually the, the idea is to bring in. The capability to recognize unknown bears as well, so we can really get a full picture on the full population.
Brian: Do they all have names at this point?
Melanie: Um, most of those bears do have names.
They all have numbers. Um, some of them have names. Um, usually they’re kind of descriptive names. Sometimes they’re named after certain geographic areas as well. And then some, some that were named quite a long time ago have some names. Names after people’s children and things like that. But we try and avoid that as much as we can now.
And we, we try and use descriptors as much as possible. But it’s helpful, you know, just to remember, it’s easier to remember a name in descriptor for an animal than it is, you know, F2700 or something like that.
Brian: Ed, uh, so we’ve been talking a lot about data. These are big data sets, especially when you’re talking about video.
Let’s talk about the hardware because we’re out in the wilderness, we’re resource constrained, battery powered. Talk to us about the hardware side of the story here.
Ed: Yeah, I mean, this is, this is one of the big challenges that these, these camera chops that are available today, they, they do an amazing job at what they do, which is to be able to trigger on something like motion and create really great video or image, uh, image data for those occurrences.
But the compute we need to run machine learning. Some of these machine learning models is quite a bit more significant. And, um, you know, most of the machine learning in the past years has been running in the cloud or on really kind of powerful, powerful devices. And companies like arm are developing I.
- That can really help accelerate this work, but at a very small cost factor. So, for example, the arm ethos you line of micro NPUs or something that we’re working with a lot of our semiconductor partners to build into solutions, which have very efficient math engines, basically that can allow the machine learning execution to happen much more quickly, which would then allow you to put the whole thing back to sleep when you’re not doing something.
So, the idea with the hardware here is, you know, you’ve got a lot of math to do to run your machine learning algorithm. But that only happens when a bear has now come by and triggered your device. You’ve now need to detect that. Okay, it’s a bear. Once I have the bear, I need to get the specific features of the bear that I’m looking for in our case of face and then try to match that face with the database of faces we have.
And then the device can go back to sleep, and You know, stop draining power, basically just get back into the mode where it’s waiting for the next motion detector trigger to happen. And so, these accelerators that are starting to show up in a lot of these, uh, Semiconductor solutions these days allow that all to happen so much more quickly in a more efficient way to where we can now just power the device back down, uh, and get longer battery life out of these types of solutions.
Brian: And we are we running the models directly on the devices or is that done in the cloud still?
Ed: So, we’re running them mostly on workstations and cloud today, but this was the whole goal for this challenge was to start figuring out one, finding more efficient models, but also get them running on a platform.
For example, like the, the NXP IMX93, which is the platform that we chose for this challenge, which has a couple of Cortex A55 processors from Arm and a Cortex M33 microcontroller. And the ethos you 65 from the family of micro neural processes that I talked about. This is a good kind of device that has that mix of, you know, low power, uh, you can shut things down or just run the microcontroller and you can use this ethos to help accelerate things.
So, it still has enough of that compute capability to run some of these models. But we really haven’t been running them in that Mode, and it does take some effort to get there. And so that was a big part of why we wanted to run this challenge was to get an idea of start, get some people familiar with that flow
Brian: and part of that flow.
My understanding is that you introduced some of the participants to virtual prototyping tools like our virtual hardware. And I, I have to assume that that’s fully embraced is going to speed up the, the Acceleration going forward now.
Ed: Yeah, absolutely. So, you know, one of another one of the reasons why he picks the IMX 93 was it is a platform that we had been working with NXP to build an Arm virtual hardware virtual platform for.
And so, what that means is we’ve got essentially a model of that hardware platform that can run in the cloud. The thing with hardware platforms is, you know, they’re a physical device. If you have 10 engineers and you want them all to work on it, you need 10 devices. You know, these engineers may be all over the world, uh, as they are in this challenge.
Logistically, it’s complicated to get the hardware into everybody’s hands. So, one of the things that virtual hardware allows us to do is the same developers can go and start developing their software algorithms and testing all of their applications. using this virtual platform version of the hardware.
It’s binary compatible with the real hardware. The same kind of image that you run on the real hardware works on the virtual hardware. And so, it basically allowed them to get started and developing much more quickly. The other big value is once you have developed this application, it gives you a way to run tests and continuous integration and the kinds of things that developers need to do to maintain their software over time.
It gives you a way to run those things in a scalable fashion. Using the cloud rather than having to build a farm, a board farm or a bunch of devices that you have to get data into and out of. So, it definitely has a lot of value for kind of squeezing the development process and getting it started more quickly and shortening the end time.
Brian: So, we’re talking about data capture and we’re talking about artificial intelligence, machine learning, Melanie, there are some ethical considerations, as I understand it, when you’re working with one of the first nations organizations that you mentioned earlier, the Nanwakolas Council, I’ve probably mangled that name, but, uh, tell us a little bit about what those considerations were and how everybody navigated those.
Melanie: Yeah. So, I mean, I think from the beginning, um, Of the Verity project, we wanted to make sure that we were developing, um, software that Was usable and that had end users in mind. And I began collaborating, um, with the first nation first member nations of number close council about five or six years ago now.
And basically, they were really interested in what we were developing and our, our ideas around, um, using noninvasive technologies to study bears. Um, at the same time, um, The guardian programs for each member nation. So, it’s who I work with directly. So, the guardian programs are basically kind of the eyes and ears for their nation on the land.
Um, they’re responsible for different aspects of stewardship within their traditional territories. So, they do everything from, uh, wildlife research and monitoring to spill response, to working with search and rescue. So, kind of anything that’s going on within their territories. They’re part of, um, so when I was speaking with them about some of the grizzly work that we started, um, at my original field site, they were really interested to get involved.
Um, which was perfect timing really, because as I say, we were starting to think about how can this work that we’re, you know, doing, how can it be applied and how can we start to see benefits to, um, the conservation of bears on more of a population scale rather than just a specific site. Um, so by working directly with the nations, we’ve been able to collaborate on not just the collection of data.
So, we’ve got camera traps now, a whole network of cameras across six different First Nations territories. Um, and so therefore we’re collaborating not only on the collection of the data, um, which we’re using to train our models, but also how that can then be applied towards aid in decision making as well.
So, as I say, the nations are interested in understanding the distribution of bears within their territories, the movements of individual bears or the movements of bears in general across traditional territories, and also, you know, specific sites or specific questions around areas that may be requiring higher levels of protection, for example.
So, by providing, um, data on individual bear movements, we can provide a bit more comprehensive information about the status of bears within their territories, which can be used to help make decisions around things like, um, development or protection. So, it’s been, as I say, a focus for us from the beginning of how we apply these models.
Um, so working directly with the nations has provided the ideal kind of. Um, test for how, how this can be applied in the real world. And we’ve tried to use that since we’ve established that collaboration, we’ve tried to kind of use that to drive forward. Um, the questions that we’re asking and how we’re going about applying the models.
Brian: That’s fascinating. Now look, you’re a scientist, so you must be like a kid in a candy store at this point with this, this technology, the data’s coming in, you went into the project with certain assumptions that you wanted to test against the data. How do you see these AI models? In other words, impacting your future work and the kinds of questions that you’re going to be asking.
Melanie: I mean, it’s been absolutely fascinating to see, to kind of be able to study the performance of these models as well and see how well they’re working and, and kind of look at our expectations of them. Um, you know, a question from the beginning that we’ve had is kind of, you know, are they ever going to be able to identify, bears with the same kind of accuracy as people, which is really a hard question because we don’t actually fully know how accurate people even are at being able to recognize these individual bears, right?
That’s just kind of the, uh, the benchmark that we’re kind of using. you know, individual ID using, using faces on a species that doesn’t have specific markings is probably one of the most difficult types of this like fine grained classification in terms of, from an animal perspective anyway. So, I feel like we’ve really kind of jumped in the deep end almost and trying to go straight to individual ID, but we did that because we thought it had real benefits for, for research and monitoring of bears.
So, um, I guess just kind of. Reestablishing what our expectations are along the way and being really able to look at the performance of these models and try and study. Um, look at like their performance across different individuals. I mean, we even have information for our, um, specific population of bears about how closely related some of these individuals are as well.
So, we’ve always had questions around, are we more likely to confuse bears? Do you know, do bears are more related? Do they look more similar? And therefore, are the models more likely to confuse them? Cause that’s something that we noticed, you know, in person doing it, that a lot of bears that we know are kind of mother offspring can sometimes look more similar.
Um, and that is something that we’ve kind of seen at least on a, from a small sample point of view in the data as well. So, just trying to fully understand as much as we can, how these models are working. Cause again, a focus of ours from the beginning has always been to try and develop robust models. So, before, you know, we don’t want to run in.
And kind of start applying the models before we’re really confident in them and that they can be applied and have a similar level of performance. The worst thing that we could do is develop models that seem to work really well in theory. And then somebody goes out and tries to use them in the field and they’re, you know, underperforming compared to what we, what we suggested.
Because as I’ve said already, these, these data can be used to make really important management decisions around wildlife. So, I think it’s really, really important that we have confidence in the models that we’re developing, and we try and understand how they’re working and what it is, you know, what it is about the bears face that is concentrated, you know, just to try and break it down as much as we actually can.
Um, to have as much confidence to then go on to apply them. 64,
Brian: $64,000 dollar question. Melanie, you’re the scientist, Ed, you’re the technologist. What’s the impact of this technology on bear conservation efforts globally?
Melanie: From my point of view, um, it’s providing the tool. It’s providing another tool, um, that can be used to study bears in an, in a noninvasive way.
You know, some of these questions that I’ve suggested that we can ask of the data. Could be, could be asked using GPS collars. So, a lot of studies will physically tag animals so you can track their individual movements. Um, but you know, there’s certain circumstances where people may not want animals to be physically captured.
And there’s a lot of different questions that go around, you know, on in relation to that. It can be quite risky for animals and for the scientists involved. It can be expensive. So, we’re trying to kind of develop a tool that is kind of cost effective, that is noninvasive, and that can be applied kind of throughout the range of, of, of bears or the specific bear species that we’re concerned with.
So, I feel like it’s providing another kind of piece of the puzzle to be able to use like image-based data to ask specific questions that in the past and without individual ID, we’ve not been able to ask. So, in places where it’s more appropriate to go out and set out a network of cameras and then leave those cameras up.
You know, you can sometimes leave these cameras for even a year if the batteries last long enough and then go and collect all that, all that data back in without having to physically be there. Um, or to be physically, you know, checking up on your animal. Um, you know, it’s, it’s incredible the amount of data that we can gather from them.
So, for me, I think the, the main kind of takeaway is that it can help us to gather more data, to be able to ask more in-depth questions, to get a more comprehensive understanding. of bears use of an area or a landscape, look at trends over time, like I say, do that in a way that can be applied and is as noninvasive as possible.
Ed: I think it’s also interesting that forums like this podcast, the AI for Bears Challenge, and some of the other things we’ve talked It just brings awareness to more, more of the technology community and gets more technologists involved in these areas, which can help us more quickly apply all of these amazing, uh, machine learning and AI applications to, you know, fundamental challenges that face the planet.
Melanie: I totally agree. I was just going to add, like, I feel like the collaboration is, is the key here because we’re, we’re all bringing our own expertise as well. And so, you know. Ed might recommend something, and I might say, yeah, but that wouldn’t work in the field or vice versa. So, we’re all bringing our own expertise and knowledge.
Which can just kind of help to drive these types of projects forward. And I don’t think we would probably either be in this position if we tried to do this, you know, independently.
Brian: And they’re not just hugely useful project. They must be fun to work on, you know?
Ed: Absolutely. That’s how I jumped into this.
As I said, you know, just. Being able to watch, uh, watch these bears at Brooks Falls. Um, you know, I’ve had a chance since I started this project also visit the site that Melanie works out in British Columbia, which was amazing. Um, yeah, it’s Watching this wildlife is, is, is really amazing. And, and when you start to understand and identify the individuals, it’s, it’s not much easier to, to really kind of get involved with their lives and understand what impact we’re having on their lives across the planet.
And so, um, yeah, I think it’s, it’s. It is a lot of fun and it is, it is, uh, it also brings a lot of good awareness for, for people.
Brian: Well, we could talk for hours. This is a fascinating topic, but you have lives and speaking of lives, what’s next for each of you, Melanie, I assume you are. Headed off into the wilds at some point soon.
Melanie: Yes. Um, actually next week I’m heading back out to, uh, to one of my field sites. So, I’ll be going out, checking our whole camera network, um, checking the batteries, pulling the memory cards, and then going through that slow process of initially processing the footage and, um, seeing if we’ve got one bear, seeing if we’ve got 10 bears on cameras.
So, a lot of the times that’s linked to what’s going on with food availability. So, I’ll be. Checking out the rivers there and seeing if we’ve got lots of salmon in the river systems. Because then that’ll mean that we’ve got lots of bears captured on camera as well. So, um, and then our season’s almost at the end for, for grizzlies, because they’re about to, to head to sleep in maybe a month and a half, maybe two months in our area.
So, they’ve still got a bit of feeding to go yet. But from what we’ve seen so far, they’re, uh, they’re getting nice and large, which indicates there’s lots of food in the rivers, which is great.
Brian: I was once in Alaska and going up a creek with a, with a guide to look at grizzly bears and the sound around a bend of a grizzly ripping apart a salmon was the most awesome sound I’d ever heard in my life.
And I thought, let’s keep our distance.
Melanie: Yeah, that’s cool. You can also, there’s a certain smell and people that have spent time around salmon spawning streams will know you can smell, even if you’ve not seen a bear and you’ve not seen a salmon, you can smell. When there’s salmon in the river and Lots of bass guts on the ground and things.
Ed: Maybe a good sign for the rest of us to stay away.
Brian: Indeed. Ed, what’s next for you?
Ed: Well, you know, I want to continue to find ways to leverage my role at Arm, uh, to, to help out this project. I think the Arm ecosystem is. Is the right kind of ecosystem to really be able to drive these kinds of technologies for, for building out these edge devices that can be intelligence and can really bring more real time feedback to, to the kind of research that that Melanie and similar researchers are working on.
So, you know, I want to continue working with, with our ecosystem and the wild labs community and potentially education groups like a fruit punch AI to. Keep spreading this word, get more technologists involved, and continue to develop the projects that really benefit, not, not just this project, but, you know, a lot of these fundamental technologies can help other researchers around the world that are looking at different, uh, wildlife challenges.
Brian: Well. This has been a fantastic conversation. I’ve learned a lot, especially don’t run.
Melanie: Yeah, never run from it.
Brian: Thank you both for your time. Thank you, Brian.
Melanie: Thank you very much. Thank you.