AI Everywhere: How it’s Achieved Efficiently
Summary
Artificial Intelligence (AI) can drive change and innovation and create new possibilities in many markets, but getting there can require a lot of power, algorithm optimization and processing efficiency. It can seem like a daunting landscape.
In this episode, Geof Wheelwright speaks with Dennis Laudick, vice president of commercial and marketing for Arm’s machine learning group, to learn about Arm’s role in machine learning and AI. The pair discuss the current trends around AI, what Arm and its partners are doing to make the AI journey easier for engineers and developers and what impact AI will have in the future.
Speakers
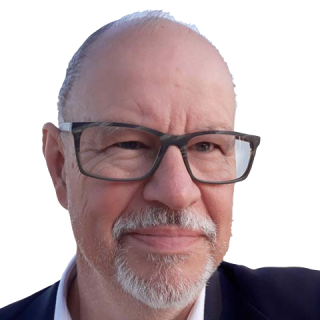
Geof Wheelwright, Arm Viewpoints Host
Geof has worked as a journalist, author, broadcaster and consultant for more than three decades – and in a variety of technical content management, corporate communications and senior management roles at several technology companies. He has contributed to a broad range of media outlets – including The Guardian, the Financial Times, The Daily Telegraph, The Daily Mail, The Independent, Canada’s National Post, Time Magazine, Newsweek and a number of specialist technology industry sites (such as Geekwire) and Travel titles (including Travel + Leisure).
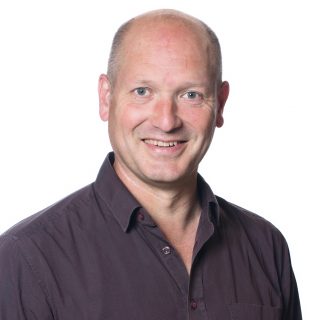
Dennis Laudick, Vice President, Commercial and Marketing, Machine Learning, Arm
Dennis Laudick is vice president of commercial and marketing within Arm’s Machine Learning Group. He is responsible for driving the commercial strategies, products and market engagements that will deliver the next wave of computing on Arm in the exciting spaces of AI and ML.
Transcript
Geof: Welcome back to the Arm Viewpoints podcast. Today we’ll be talking about machine learning and AI in an episode we’ve called AI everywhere how it’s achieved efficiently. We’ll discuss the current trends around AI, what arm and its partners are doing to make AI more efficient, and what impact AI will have in the future. With me today is someone who has a deep appreciation for what machine learning and AI can achieve. Dennis Laudick, Vice President of commercial and marketing within Arm’s machine learning group. Dennis has more than two decades of experience in the mobile, automotive and consumer electronics industries. Prior to joining the machine learning group, he led all of the business and partner engagement aspects of arms GPU business. Welcome, Dennis.
Dennis: Hello, Geof, thanks for having me,
Geof: Maybe we can start by if you could tell us a bit about arms role in ML and AI,
Dennis: Arm kind of underpins most of compute in the modern world. And one of the things that that people might not appreciate is because of where Arm sits in the supply chain. So we make designs which are then implemented into silicon, which then they implant into devices, which then are, are shipped to consumers, we actually have to be thinking about technology many, many years before it’s going to be relevant to the average person. So we’ve got a long history of looking really far ahead. If you look at some of the technologies that we’ve developed, that become really popular in the market, they’ve often started 10 years before they end up being common in the market. That’s similar to our what we’ve done with AI. And around machine learning. We started thinking about it years and years and years ago. And in fact, you could see the fruits of all that, that early thinking come forward, you know, several years ago, when we started producing CPUs, designs that had specific improvements to enable more and more AI. So dot product instructions, more vector capabilities, that kind of thing. So that said, we kind of see AI as a massive shift, fundamental shift in technology. So to that end, we see it affecting pretty much everything that we do in one way or another. And so you can see that coming out in all of our products. So we’ve been producing CPUs for several generations now that have had machine learning, targeted improvements. Our GPUs similarly have been getting better for machine learning over the last few years, but also a lot of investment into the software tools that you know, enabling an ecosystem helping develop standards to make the technology accessible. So, so yeah, we’ve been working on it for a long, long time.
Geof: Maybe we can talk a little bit about the results you’ve actually achieved in the market. I mean, some of them you talked about, but maybe the broader impact around both AI and machine learning?
Dennis: Yeah, absolutely. Um, so as I said, you know, we’ve been implementing improvements for machine learning, targeted machine learning, and in most of our products, or at least many of our products over the last few years. And I think it’s been kind of interesting to be in this space, a lot of the early dialogue around AI, you know, several years ago was somewhat dominated by products that were specifically targeted at that. And so you had a lot of talk about, you know, processors and different algorithms and so forth, which were dedicated to doing machine learning. The reality of the matter is that while all that was going on, and certainly that was applicable to certain parts of the market, the rest of the market, the vast majority of it was just getting on with implementing AI. They weren’t waiting for any hardware, they were just doing it. And so a lot of the AI and ML that’s happened today, certainly outside of the cloud, has been happening on devices. And I’m very comfortable in saying that the majority of AI which runs outside the cloud actually runs on an Arm device, of one form or another. So, you know, one of the great fallacies is that you need this dedicated, dedicated hardware, and certainly there are places for that, but a lot of AI and machine learning will run just fine on it on a CPU today, you know, things like basic language processing, a lot of image object detection, things like that. They can, depending on your performance needs, they can often run quite well on a CPU or GPU. And as we make our products more capable in that area, we see people taking advantage of that straight away. So the odds I’d go as far as saying the vast majority of AI outside of the cloud today actually already happens on Arm. It’s just something that’s quietly happening, a quiet revolution that’s occurred over time.
Geof: That’s pretty staggering. So you’ve been at the heart of this. So how have you seen the trends around AI changing, and is that that AI hype cycle over?
Dennis: The hype cycle questions when I find really, really interesting. I mean, obviously, part of my, my responsibility is looking after strategy. And so I’m always kind of thinking, where are we in the journey? And I’ve been through several hype cycles before. And, you know, they, they, I certainly believe that I’ve seen it happen multiple times. In AI, it’s been a little bit different. I’ve actually seen two different cycles happening in parallel, there’s kind of the, the public cycle, and then there’s this sort of technology cycle. So on the public cycle, you know, I think we definitely have crested the hill in terms of the technology, you know, there was five years ago, a lot of the discussion was dominated by dystopian views of Terminator robots and, and killer drones and things like that, you know, where we’re about to just be supplanted by AGI. And, you know, I think most technology people at the time, understood that that wasn’t necessarily where we were going in the short term, that wasn’t what we were enabling. So from a technology standpoint, though, we did know that we were enabling a whole new wave of capabilities we’ve been struggling to get to in computer science, you know, perception, things around being able to deal with complex objects and around language and things like that we were struggling to create algorithms complicated enough to be able to deal with this. But, you know, suddenly, we had a tool for doing this. And in fact, we had a universal tool, we had a universal function generator. And so that created a fantastic shift in technology. But it also had a lag to it in terms of the, you know, the skill sets that we needed to develop as engineering communities and the tools we needed to develop to help us automate the processes and make them more democratized and accessible. So from that perspective, I think I’ve only seen it build and build and build, it hasn’t really gone into any sort of retreat. And in fact, I would say, we’re just coming into the springtime of what we can do from a technology perspective. People in the engineering communities now more retooled to be able to take advantage of this capability and understand it, the tools are becoming to the front that are allowing us to automate a lot of the really complex processes and we’re starting to understand what we can do with this. And we’re starting to get out of some sort of initial use cases that were very popular and starting to propagate it into more and more things, which are affecting our lives around healthcare, security, and so forth. So, so yes, I think the public hype cycle is probably crested, you know, I’ve looked recently at where, I think Gartner were on their hype cycle and I think it’s probably just about right. But from a technology perspective, it doesn’t seem to be going into any trough, it just seems to keep building and building and building.
Geof: Right, so we’re avoiding that trough of disappointment.
Dennis: From a technology perspective, I actually think we are, I did somewhat try to understand if it was coming, but I think we’re just going keep growing from here, I think we’ve kind of been through that, what would have been that phase, and it’s that’s been more of a maturing phase, rather than a trough, from a technologist perspective.
Geof: Well, and that actually kind of brings me to an interesting point around, as AI evolves, it looks like you’re starting to address some other key issues like efficiency and sustainability. So what’s Arm doing in particular, to enable AI to become more efficient?
Dennis: Yeah, efficiency, again, is one of those really interesting ones, and particularly at Arm where, you know, everything we do is kind of about efficiency. And it’s really kind of our, our bailiwick in the market. Before I go into the technology side of it, I think it’s worth kind of stepping back. And taking a look at the topic of AI and power in general. Again, along with that, a lot of the dystopian views, there was a view that AI was going to consume the world in terms of power, you know, we suddenly had a universal function generator, the capabilities were unfathomable. And so the demand for being able to do more and more of this and to create more and more complex functions or capabilities was it’s just been insatiable, you know, we really haven’t reached the end of it at all. So, you know, much like the predictions about the internet in the 1980s, there’s a lot of question around whether or not the AI is going to consume the planet. There was an interesting paper at the University of Massachusetts Amherst, I think, in 2019, where they did some calculations around training of some rather large networks like a transformer. I think they came up with something like 300 tons of carbon just to train one model. So you know, I think when you see those kind of numbers you can worry that the machine learning is going to gobble us and you know, the insatiable demand for it’s going to be a concern, but I think, again, we’ve been through an early sort of a frenzy phase around that, and I’m also seeing now that there’s going to be, there’s a lot of benefits coming to AI a huge number of benefits. I mean, some of the some of the things we’re seeing now is people have gone out of, you know, an early phase where everyone was just going from more complexity and more capability. Now, people are starting to look at efficiency in the networks that enable machine learning and AI. And we’re seeing some astounding numbers, order of magnitude type efficiency improvements. But more importantly, I think it’s the application of AI has the potential to make almost anything more efficient. For example, you can look at data centers, you know, data centers are being built, largely to a significant degree to support AI. But AI is also being used to control those both within the data center, and you know, how traffic and processing is distributed from one location to another as their populations demands change. So, you know, some people have seen improvements, energy efficiency improvements around 25%, in the data center, just using AI methodologies. Some other things we’re seeing, you know, one of the big trends we see at Arm is that a lot of AI is starting to move now out of the cloud out onto devices, we’re seeing AI move to the edge in almost every industry, you know, it early development was done in the cloud, that was kind of the site the lab part of it, but now that people are, are starting to distribute it, or apply it to more areas that is starting to go out and to the edge just for various reasons. You know, I think one large tech company coined the phrase the laws of physics, the laws of economics, and the laws of land, referring to the inability to ship that much data to the cloud, the poor economics and being able to do that and build the data centers to centralize the world. And this the privacy and security aspects of it. As the AI moves out to devices, of course, you save a lot of power in terms of transmission and you know, on the edge, we can do things incredibly efficiently. So again, I see AI kind of as a distributes becoming more power efficient. But also, you know, AI can be as I said, it can be used to make almost anything more efficient. So, thermostats, pumps, you know, transport logistics, the amount of fuel that you can save, by having more efficient transport, it’s just affecting almost everything so I’m actually really optimistic about the impact AI is going to have on us in the world. So that’s kind of the big picture looking in at our technology. And a lot of times people ask me, how does arm get to the power efficiency they get? Why are they, you know, what do they do that makes them the power efficient processing technology? And it’s an interesting question I’ve been asked many times over the years at Arm, and I kind of equate it to asking a question, how is the brain power efficient? You know, the brain is one of the most power efficient processes on the planet? How, why, what’s the thing that makes that efficient, and of course, it’s not one thing, it’s, it’s a huge number of things. You know, it’s kind of a, it’s something that’s central to the design. So so there’s just, we take a sort of efficiency, first view on everything. It’s not about performance versus about efficiency first. So with that in mind, we implement a lot of different things in the technology there. You know, for example, we look at machine learning very much as a data problem before we look at it as a processing problem. Machine learning involves vast amounts of data moving around. And so anything you can do to minimize the data movement, whether it’s, it’s reducing your networks, adding compression, minimizing your needs to reload data again, and again, all these Can, can make your system more efficient and your technology more efficient. But also, we implemented, as I said, a lot of different things across our tech technology, it’s not about one thing. We do a lot of different things.
Geof: So Dennis, you talked about data centers and the tremendous energy efficiencies that can be achieved there, and you alluded to a couple of possible other examples. And I’m wondering if we could talk about more broadly, examples of companies using AI to become more energy efficient?
Dennis: This is this is an area find a really exciting, there’s, you know, AI and machine learning, we’re seeing it go almost everywhere, in terms of the devices that they use arm technology. And so with each one of those, as I said, you could, you can imagine almost anything that consumes electricity, being able to be made more efficient using AI. So if we look at this as kind of like a few good examples of drawing from the consumer industry. In the first case, if you look at refrigerators, we’ve got a partner in Turkey called Arcelik and they’re one of the major appliance manufacturers in Turkey, and they’ve done some really interesting experiments. So according to their analysis, refrigerators are the second largest consumer of electricity in the home, accounting for on average, globally around 13% of the total energy consumed in the home. And what they did was they retrofitted a some refrigerators with some really small reinforcement learning algorithms that were running on a very small device, I mean, something quite small in terms of processing capability. And just using that machine learning, they were paying attention to the behavior of the environment that the refrigerator was working in and learning how the family or the environment would behave. And using that to make optimizations in terms of when the power of the pump would turn on and off, and how it was using power. And just using that they were able to reduce the power consumption of the refrigerators by about 10%. And this was existing refrigerators. Which if you extrapolate that globally, it’s wow, that’s it’s a huge number. So I think the calculation they came to from that was, if you were to just extrapolate that and use that widely across Europe alone, you’d be able to shut down something like nine power plants which is just incredible. So you can imagine the impact of that going globally. And that’s just looking at refrigerators. So apply that to all the other devices in your home. If we then switch over to the industrial side of things, the industrial industry is really important to us. But it also consumes a lot of power. So pumps are a good example, that I think is reasonably central to what I consider to be power, power consumption. Pumps are responsible for something like 10% of the world’s electricity usage. And there’s an estimate going around that 90% of those are inefficient and that they can somehow be improved in one way or another most often by some form of AI. Either being aware of the environment and using them more efficiently for that application, or you know it through to being able to do already predictive maintenance based on vibrations and AI analysis. Grundfos, they believe that if we were to use AI techniques, just locally applied, it’s very difficult to do AI for on all industrial pumps over a remote connection. But if you were to do those locally, they estimate that you could reduce the power something like 15 to 20%. Which is just incredible. And if you were to extrapolate that globally, I think it turns into something like a 2% Global savings, which is just astronomical. Just again, looking at that, that reasonably narrow application space. And again, you can apply it to almost any type of industrial application that consumes power. And then you know, more widely it, like I said, affects everything transport logistics, being able to save fuel data centers, 5G networks. Yeah, it’s almost anything is said the consumers power has the potential to get more efficient.
Geof: Right. And that’s probably a good point to reflect on the future. So maybe with our final question, we can dive into how you see AI changing in the future, and the impact you see it having in the next few years?
Dennis: Yeah, so its mind blowing, in terms of what this technology can achieve. You know, I’ve heard too referred to as software 2.0. Industry 4.0. I don’t think that’s understating it. One of the engineers in my early early days working in this in this role said, right, go to go to your favorite search engine, look up your favorite computer science terminal term, and add machine learning to and you will see people who were people who either tried or there’s research on that, and I did that a bit. And they were right. This year, machine learning has the potential to go almost everywhere. And I think it is slowly going to revolutionize the entire technology space. I don’t think that’s an overstatement. You know, we now have where we were developing more and more complex algorithms to try to deal with more uncommon and more complex situations, we now have a universal function approximator that is awesome and immense. So I see this really getting into every part of our lives. Some of the areas I am most excited about our health care, for example. You know, there has already been early research in using health related images and machine learnings ability to more accurately diagnose what’s going on with those. Machine learning is really good at trends and statistics and a lot of understanding ailment. And so forth has to do with with understanding the, either multiple factors and how they play into a trend or, or, you know, making sense of complex data. You know, and even down to individual devices, you know, fitness trackers, I use a fitness tracker, I can see numerous applications for machine learning to improve many of its functions. So I think healthcare is going to be incredible in terms of its impact, power efficiency, you know, some of the examples I gave, I can see, just almost everywhere that power is consumed today, we can we can have efficiencies, improvements, so I can see huge amounts of power improvements in motors, engines, electronics, UIs are another area I think are going to become incredible. You know, the way I tend to look at it is a lot of the, the early stages of the electronics industry was around how we could create user interfaces that were easier for humans to interact with. And you could arguably say the smartphone era was fueled by that to a large degree. But I think the AI era is going to be fueled by user interfaces that make it more natural for us to do the interaction. So they’re going to do the work to interact with us, rather than us having to learn them, they will learn more about us. Some more natural user interfaces, be a voice, gesture, presence or anything like that. Transportation. As I said, fitness trackers, you know, every step of the semiconductor manufacturing and design and manufacturing process is being improved using machine learning, in one way or another. So, so yeah, I think almost everything around us will just quietly get better. You know, as I said, at the near the beginning, I think you went through the I tend to look at technology and phases of sort of discovery, maturity and production. And I think we’re just into the maturity phase, where we’ve finally got the people that understand the technology, that now we’re coming out of just a few sort of centralized use cases. And we’re starting to see people implement this even in tiny sensors. You know, a little bit of machine learning next to a sensor makes the data coming out of that sensor much more clean and much more usable. So yeah, I think this is going to go everywhere. I think, you know, the next phase really is about maturity and democratization. We’re putting a lot of effort into tools and standards and helping people understand bringing people together. Yeah, so I think you know, it’s just going to be immense. This is going to make our lives different quietly in the background, but it’s going to change everything for the better in one way or another.
Geof: I really appreciate those insights, Dennis, and I’m sure our listeners will as well. We look forward to bringing you more news in the next episode of Arm Viewpoints and we look forward to connecting with you all again soon. Thanks for listening today.