How Ampere is Shaping the Future of Data Centers and AI
Summary
As the demand for computing power continues to skyrocket, so does the challenge of balancing performance with power efficiency. On the latest episode of Arm Viewpoints, host Brian Fuller sits down with Jeff Wittich, Chief Product Officer at Ampere Computing, to explore how Ampere is tackling some of the most pressing issues in modern computing.
Founded in 2018, Ampere Computing has disrupted the market with its innovative ARM-based processors, designed specifically for cloud and edge environments. Wittich shares how the company’s mission—delivering high-performance, power-efficient compute solutions—has positioned it as a trailblazer in an industry dominated by x86 architectures.
Key Takeaways from the Podcast
- Rethinking Data Center Efficiency:
Wittich explains how Ampere’s processors address the growing power consumption in data centers by designing CPUs that are not only faster but also far more efficient. These innovations are critical as workloads like generative AI and video processing demand ever-increasing performance. - Generative AI and Processor Design:
The explosion of generative AI has transformed the way Ampere approaches processor design. Wittich delves into how Ampere’s general-purpose CPUs are optimized for modern workloads, including large language models and inference tasks, enabling scalable solutions for AI-intensive environments. - Ampere One and Ampere Aurora:
Wittich introduces Ampere’s flagship processors, Ampere One and Ampere One Aurora, which are setting new benchmarks for performance, flexibility, and power efficiency. He highlights unique features like fanless designs that enable deployment in challenging environments, including space. - AI at the Edge:
The conversation also covers the increasing shift of AI workloads to the edge, driven by the need for low latency, enhanced privacy, and localized processing. Ampere’s CPUs play a crucial role in enabling efficient and scalable edge computing solutions. - Real-World Impact – SpaceTech Case Study:
A standout example of Ampere’s success is its partnership with SpaceTech, where its processors delivered a 2.6x performance improvement while reducing power usage, proving their capabilities in real-world scenarios.
Why You Should Listen
This episode of Arm Viewpoints offers a fascinating look into the future of computing, highlighting how Ampere is bridging the gap between innovation and sustainability. Catch the full episode to learn how Ampere Computing is shaping the future of compute environments—from data centers to the edge.
Call to Action:
Listen to the full podcast on Arm Viewpoints and discover how Ampere is powering the next wave of technological innovation!
Speakers
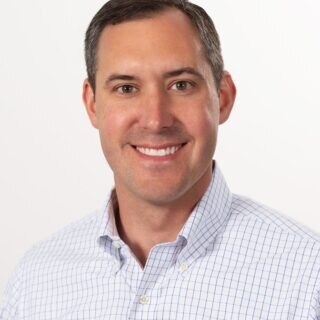
Jeff Wittich, Chief Product Officer, Ampere Computing
Jeff Wittich is a seasoned technology leader with over 20 years of experience in the semiconductor industry. As the Chief Product Officer at Ampere Computing, he drives the vision and strategy behind Ampere’s cutting-edge ARM-based processors, designed to deliver sustainable and efficient solutions for cloud-native and edge workloads. Under his leadership, Ampere has become a trailblazer in revolutionizing data center performance with innovative architectures that balance scalability, power efficiency, and security.
Before joining Ampere, Jeff held senior leadership roles at Intel Corporation, where he was instrumental in developing five generations of Xeon processors and growing Intel’s Cloud Platform Business revenue sixfold. His expertise spans product development, market strategy, and aligning cutting-edge technologies with evolving customer needs.
Jeff’s relentless focus on innovation has helped redefine the semiconductor industry, ensuring processors meet the dynamic demands of hyperscale cloud computing and AI workloads. Today, he leads a world-class team at Ampere, pushing the boundaries of what’s possible in sustainable, high-performance computing.
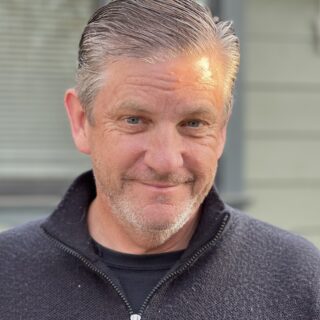
Brian Fuller, host
Brian Fuller is an experienced writer, journalist and communications/content marketing strategist specializing in both traditional publishing and emerging digital technologies. He has held various leadership roles, currently as Editor-in-Chief at Arm and formerly at Cadence Design Systems, Inc. Prior to his content-marketing work inside corporations, he was a wire-service reporter and business editor before joining EE Times and spending nearly 20 years there in various roles, including editor-in-chief and publisher. He holds a B.A. in English from UCLA.
Transcript
Highlights
- Ampere’s Vision and Evolution (01:00)
Ampere’s founding principles to revolutionize data centers with power-efficient ARM architecture and insights into its rapid growth since 2018. - Addressing Power Efficiency in Data Centers (03:00)
The increasing power constraints in modern data centers and how Ampere tackled inefficiencies with innovative CPU designs. - Generative AI’s Impact on Processor Design (07:00)
How advancements in generative AI, particularly transformer-based models, influenced Ampere’s strategies for general-purpose CPU optimization. - The Ampere One and Ampere Aurora Explained (18:00)
A deep dive into the features, applications, and power efficiency of Ampere’s flagship processors, including fanless designs suited for edge deployments. - AI at the Edge (22:00)
The growing shift of AI workloads to edge environments for better latency, privacy, and data management, including Ampere’s role in enabling this transition. - Case Study: SpaceTech Deployment (26:00)
A real-world example showcasing Ampere’s processors powering edge AI solutions for SpaceTech, achieving a 2.6x performance increase over previous x86 solutions.
Transcript
Brian: [00:00] Welcome to Arm Viewpoints. I’m your host, Brian Fuller, and today we’re joined by Jeff Wittich, Chief Product Officer from Ampere Computing. Ampere was founded in 2018 by Rene James, a former Intel president, who had a bold vision, revolutionize data center computing using the Arm architecture. In this episode, We explore how Ampere is addressing the mounting challenges of power consumption and performance in modern computing environments from edge to cloud, the origins of Ampere and its strategic decision to build Arm based processors for data centers, how generative AI has impacted Ampere’s approach to processor design and the distinct requirements of AI training versus inference, the evolution of edge computing and why certain AI workloads are naturally gravitating towards edge computing.
Deployment [01:00] Ampere’s product strategy, including innovations in chip architecture and AI acceleration. A real-world case study of Space Tech’s implementation of ampere processors for Edge AI applications, and much, much more. So now we bring you Jeff Witt. So, Jeff, welcome. Thanks for taking the time. Yeah, glad to be here.
Thank you very much. Catch us up, if you will, on Ampere, which burst onto the scene. I don’t think that’s too hyperbolic in 2018, right? You Rene came out of Intel and we’re going to, we’re going to attack this particular market but not with an x86 device. We’re going to attack it with the Arm architecture.
Take us back there briefly and then bring us up to speed where you guys are today.
Jeff: All right. Perfect. Yeah, you’re right. You know, especially in its [02:00] CPU time scale, you know, six years is nothing. So, you’re right. We did kind of burst onto the scene six years ago. We’ve done a lot since then. So, kind of to go back to you know, 2018, 2019 you know, what are our real vision was, you know, when we looked out across the data center landscape, Okay.
We saw a couple of key challenges that were emerging. One was power. It wasn’t as obvious six, seven years ago that this was going to be a massive constraint. You know, to put it in perspective, for about 15 years there, the total power consumption in the US never went up. We had many, many years of very consistent power consumption.
And so there was always spare power on the grid. And that was because we had created all kinds of efficient ways to reduce the amount of power, even while new usages were coming online. Well, a couple of things were starting to change. One, vehicles were electrifying. That’s a really good thing, right?
Takes things away from using fossil fuels in our vehicles, but it also does mean that There’s an increased demand on the, on the [03:00] grid. And the second thing is that there were factors that were making it clear that data center power consumption could start to increase, even though it hadn’t increased for a long time.
The things that were happening there were, you know, workloads were starting to change. Workloads were becoming more and more compute intensive. This analytics workloads got more sophisticated. As the AI Workloads of the time, which were more computer vision and recommender model focus, even those workloads were getting more sophisticated.
Obviously, since then, we’ve seen, you know, gen AI And LLMs Really just ratchet that up by another order of magnitude. So, workloads were getting more compute intensive. We’d already kind of used up the easy power efficiency gains. No data centers are already very, very power efficient. The most efficient data centers in the world.
Maybe you only have five or 10 percent waste and that’s, you know, that’s a change that maybe used to be 50 percent waste, 60 percent waste a decade ago. So great job making data centers more power efficient, but it also means there’s only 10 percent [04:00] more to go and attack. There are almost no efficiencies left there.
So, we did a great job there. And then also just the x86 CPUs at the time, they weren’t increasing in efficiency. They were increasing modestly in performance gen over gen, but oftentimes that performance gain came with the exact same gain in power. So, performance per watt just wasn’t increasing. What that meant was that Each generation at the rack level, even though each CPU had more performance, the performance, the rack level wasn’t going up.
It just meant that every single year when you put the new generation of, I’ll just say Intel CPUs in there, it meant that you had less CPUs in the rack, but the same exact Performance that you had the year before consuming the same amount of power. So, it wasn’t helping anybody create a more dense or higher performance data.
And so, we looked out across the landscape, and we said, there’s a clear opportunity here. The scale of compute continues to increase. The [05:00] cloud is obviously the model of computing that everybody’s migrated to. Whether it’s on prem or public cloud or hybrid, it doesn’t really matter. It’s a cloud-based model, which is big compute at scale.
And there, and there were clear problems that were going to make it difficult to keep scaling the cloud if the industry didn’t have other solutions. So. That’s where Ampere came about is we decided there had to be a better way to deliver high performance compute and not deliver high performance compute that was also very, very high power.
There was a way to go in and re architect things from the very most base level. And build something that was high performance, but also very low power and power efficient, and that’s where we came in. And so, we started with a very different approach than the x86 vendors. You know, we started with arm-based CPUs and really innovating all the way down to the architecture and micro architectural level to build something that [06:00] was really well suited for the cloud environment of today.
Versus the legacy environments that those x86 CPUs were built for when they were first built 10, 20, 30 years ago. So that was, that was really the impetus. And we’ve done a lot in the meantime.
Brian: You mentioned a couple of the prevailing workloads then when the company was launched around vision, generative AI literally exploded on the scene two years ago, has that had an impact on.
How you think about building solutions or is your CPU forward approach just naturally fit into the evolving demands of gen AI?
Jeff: Yeah, I think there’s two elements of it. I think at a strategic level, it doesn’t change a whole lot because. Gen AI really just made those strategic imperatives more important.
The idea that you need more and more performance, but you can’t [07:00] consume more power to do so. You’re going to run into some real constraints. So, it, it fits into the exact same space. And obviously building a general-purpose CPU means that you’re building for a lot of work. Now, five, six years ago, the workloads that I cared the most about were it was web servers, it was databases, it was video encoding.
It was AI inference, but it was AI inference in terms of. Computer vision or natural language processing. It was recommender models, non-transformer-based AI models, but that was a big focus for us back then. What’s changed is that the balance of workloads has changed a little bit. And so, a higher percentage of the workloads are now AI inference than, than before.
And the nature of those AI inference workloads has changed a bit, you know, with the transformer-based approach. And the LLM that came from that, what it means is the models are much larger than they used to be. And some of the compute elements that are best [08:00] that are best utilized for them look a little bit different than, than what the compute elements look like for the models of the past.
Now, the good thing is that’s what a general-purpose CPU is really good at. It’s really good at being really versatile. So as workloads change, it does a pretty good job at virtually any workload. But since we knew that AI inference had the possibility to be One of the predominant workloads in the future, but we did do specific things to ensure that AI inference as a workload ran really well on our processor.
Things like ensuring that all the numerical formats that people care about are, are natively supported. So, things like bfloat16 or int8. We did a lot of work on just ensuring that the performance of the hardware itself could be exposed really easily to the end user. About three years ago, we acquired a company on Spectre that was building software acceleration libraries [09:00] for AI inference.
And the result of that was that it became very, very easy for people to run AI inference on our CPU and to harness the SIMD units and the other micro architecture elements of our processor that are really good at AI inference to really get the maximum possible performance out of those. Without the end user needing to actually do anything to optimize their code or optimize their model or worry about what the framework, all that stuff just happens under the hood.
I would say that we, we foresaw some elements of this. I can’t say that I could have predicted exactly where we were going to end up today with these LLMs, but you could see what direction things were going. And so, you could start to build things into the CPU and then build the ecosystem around it so that it was as easy to run AI inference as any other workload on these processors.
Now, that being said, as we look at the very, very largest models, you know, as we get out into the multi hundred billion parameter models, the trillion parameter [10:00] models that’ll, that’ll soon come, you know, at that point, that does require some additional processing elements within the, within the SOC. And, and that’s why we, we announced a couple of months ago, our Ampere One Aurora line, which still uses the goodness of our really efficient general-purpose cores.
But then adds in some acceleration elements, not in the cores themselves, because you don’t necessarily want to burden the cores with these types of compute elements, but tightly coupled to the cores across the mesh within the SOC. So, you get that low latency, but you also have a lot of flexibility with how you scale those compute elements.
And you don’t have to make a lot of tradeoffs between whether you want to optimize for general purpose or AI inference at any given moment, given that our customers don’t know that. And they will always have to be able to balance between the two of them. So, I think that the details have changed, the tactics change over time as the workloads are changed, but the strategy isn’t different.
The strategy is still really high performance at really low [11:00] power across a flexible set of workloads, like always ran in the cloud. Those workloads just change, and that means that the compute changes with it over time. And if you can build a really flexible platform that’s able to easily change over time, integrate things into it, that Become, become general purpose over time, then you, then you have a big advantage.
Brian: I have so many forward-looking questions for you, but we’ll get to those right now at this time slice. This is a perfect question to ask of the product guru. What are the, what are the challenges around implementations that you’re hearing from customers and developers out there and how is Ampere addressing these?
Jeff: Yeah, I, I think that you know, there’s a couple of elements to that. One is that a lot of these solutions are very, very complex today to implement. The solutions are look at the platform level, look a bit different at times than the solutions at the platform level that existed [12:00] a couple of years ago.
There’s a lot more elements. System level optimization is a lot more important than, than maybe it was a couple of years ago because of the amount of data traffic, the movement between different elements within the server. One thing that we’re doing there is we, about a year ago we created the AI Platform Alliance.
The idea here was there’s a lot of people out there that are building really, really cool elements that can go into an overall AI solution. We’re building a CPU, there’s people that are building accelerators that are really good at, you know, maybe it’s different types of models, different size models, different deployment models so wide variety of accelerators out there.
Also, there’s a wide variety of ISVs that are, that are building their own frameworks that maybe sit on top of some of the, the other AI pieces to make it easy for enterprises to deploy. At a higher level, you have S. I. S. O. E. M. S. That are building a wide variety of systems that may look very different than the systems that [13:00] people were deploying a couple of years ago.
So, it’s complex. You know, there’s a lot of different players in this market, and the solutions look really complex from an end user perspective. So, it could be easy to just default to the status quo, you know, whatever the known quantity is. So, we created this AI platform alliance so that we could bring all those folks together, collaborate on how our solutions work together, and then build a set of solutions that are easy to deploy for say an enterprise AI user for a specific use case without them needing to piece the entire solution together themselves.
So easy to deploy solutions. Like for instance, we built a solution with Netting has their video processing units. So, you think of a use case like Whisper, so using Whisper for, say, doing transcoding or translation, and Transcription of live video, for instance, like a, like a newscast, you want to close caption it.
Maybe you want to close caption it in 30 different languages. Well, Whisper does a great job of that. And so, when you take an overall solution that has [14:00] NetInts, VPUs and Argental purpose CPUs and, and then you build it out at a platform level with someone like SuperMicro. Now you have almost an appliance that somebody could deploy.
So, if you’re a broadcaster, you have a box that does exactly what you want. That’s going to be able to, say, process hundreds of video streams simultaneously and translate and transcript them real time. And you don’t have to worry about piecing this together yourself. And so that, that’s one of the things that that we’re helping to address is just the complexity of the solutions is very high.
Because we are in the early stages still of, of this AI cycle. It may seem like we’re far into it. It depends on kind of, I guess, where people have been in their place in the industry over the last, you know, five or 10, 15 years, but we’re still really early in this, in this AI side. And so, we’re dealing with a lot of nascent technologies and solutions that are just coming together, you know, real time.
So that’s the, you know, the AI platform Alliance helps address that, that issue. The other issue is, is just, again, it [15:00] goes back to the power issue. There’s, there’s suddenly potentially extra more increase in compute demand. We didn’t suddenly get more power in these edge locations. You’re still constrained to maybe a hundred watts.
Your big data center is still constrained to whatever the power capacity got off the grid. In five or ten years, that can change. Obviously, we see people going in and looking to, you know, spin up nuclear reactors again and, and things like that. But that doesn’t happen overnight. That’s not a next week. Type of thing we still have a big build out that that’s going to occur before all that stuff comes online.
And while we’re doing all that and bringing that online, you know, the compute dance and keep going up and going up and going up. So, we need to do everything we can. We need more power sources. We need cleaner power sources. We need more efficient data centers. We need more efficient processors. We need more efficient solutions.
And we all that stuff to come together. And I think that you know, along with the power challenge, the thermal challenge is, is difficult to, you know, there’s a lot of cool, innovative technologies out [16:00] there direct liquid cooling, immersive cooling, some are more practical than others, and, and they can be really efficient.
But if you have a data center that you just built a couple of years ago, and you’re, when you built it, you were planning for a 10, 15, 20 year, you know, life cycle. You weren’t planning on going in and retrofitting the whole thing in a couple of years. Your kind of, you have what you have, you have the investment that you made.
These data centers live over a very long lifetime and it’s not trivial to go in and gut them or completely overhaul the architecture of them or redesign the way the racks are laid out or create new power and cooling delivery systems. Those don’t happen overnight. And it’s important that We deliver solutions that can be deployable today, everywhere at scale.
And not just solutions that eventually will be able to be deployed everywhere at scale in five or 10 years. So those are some of the problems that we’re helping, you know, people to, to solve today is just make things simpler, help them fit into [17:00] their existing environments today, because that not everything can change overnight and build and build a path to the future with these customers.
And, and I guess as, as enterprises are coming online and, you know, running more and more AI Workloads and more and more of those AI Workloads are very, very critical to them, and they want to have some control over where and how they run them. You know, it just magnifies the problem a little bit more because this isn’t just a couple of people.
Big hyperscalers running AI workloads. This is hundreds, thousands, tens of thousands of enterprises where AI is now a critical workload, and they have to figure out how to adopt this at scale as well.
Brian: So, the crown jewels at this point are Ampere One and Ampere Aurora. Give us a compare contrast and somewhere in my research, and now I can’t find it in my questions, you’re enabling fanless designs.
I think, is that? Is that right?
Jeff: We are. Yeah. So, if, when you look at it, you know, [18:00] Ampere One is our flagship product today, general-purpose compute. Today, the CPUs have 192 cores, we’ll be releasing a 256 core CPU soon. And that’s, that’s really what we see at the workhorse within the data center space, but also out into the, out into the edge.
So really efficient processors can run any workload you throw at it. And that solves the bulk of the, the data center. And you mentioned the fanless designs. Again, the type of efficiency that we’re delivering in the data centers equally applies out to the edge. You know, different scale. Maybe instead of a 500-watt server, now we’re talking about a 50- or 100-watt device that’s sitting out at the edge somewhere.
And some of those devices do need to be fanless. You know, a, a good example of one of these is we have a deployment in space today. Fans don’t work in space. Space is a vacuum. So, a fan does no good in space. There’s no airflow in space. And so those are devices that have to be passively cooled because you don’t have any way to actively move air or, you know, a liquid’s not feasible in that environment [19:00] either.
And so, yeah, we’re, we’re enabling really efficient solutions out there where you can still get a couple dozen cores, 40 Watts. Which doesn’t require any active cooling at all. That’s Ampere one is, you know, wide range of core counts, wide range of use cases, wide range of, of power consumed, but always efficient and matched to the environment that that people are looking to deploy it in.
And then Ampere one Aurora is the next step. In our, our product line, it’s taking the flexibility that we built into Ampere one where we moved to, you know, we have a chiplet approach. We designed our own disaggregation architecture, we designed the die-to-die interfaces and architecture, but we designed them in a way to be very, very flexible.
Now, the way I would maybe describe it is Ampere one uses that flexibility for us to build a bunch of products that that are flexible for us to build and to provide to the market. With Ampere One Aurora, we take that flexibility, and we start to [20:00] utilize it in different ways. So now that same flexibility that allowed us to use a lot of different chiplets to build general-purpose CPUs, you can now use that same type of flexible architecture to now start to drop in AI accelerators that aren’t general purpose.
But which seamlessly mesh with the general-purpose CPU cores. Taking that a step further it could be any work. It doesn’t need to be just AI. Now, obviously that’s the, that’s kind of the killer workload of the moment, and that’s where it makes the most sense to go in and apply those, those resources and build a specific type of accelerator for, but you can build this acceleration in for any type of workload, and this doesn’t need to be Ampere.
Developed IP or Ampere developed chiplets either, this can be third party IP that has been developed by another company. Maybe it’s something very unique to their workload or their environment, or maybe it’s something that’s incredibly sensitive for them where they don’t want this IP out [21:00] there and deployed by other people.
So, so Ampere One Aurora kind of takes that flexible framework and it now really takes. General purpose compute to another level because we’ve created another way to create that general-purpose aspect while also having acceleration. So, you kind of don’t have to in a way you don’t have to choose anymore between whether you want something that is general purpose versus something that is domain specific, you can actually have.
Any of that within a, within a flexible set of solutions that can go into the same types of platforms. And so, it’s really, I think it’s that evolution of compute where maybe general-purpose compute has now turned into AI compute and AI compute is that broader set of workloads. Some of which are very specific and some of which are very general.
You talked earlier
Brian: about power at the edge, but because you sit in such a unique position, you have great [22:00] visibility. About what’s going on at the edge, there’s a movement to move more AI compute to the edge, keep it there for reasons of lower latency for privacy and security. How do you see in the next couple of years that evolving?
There are obviously some workloads I’m thinking video files are better suited being computed on in the cloud. But how do you see edge workloads evolving?
Jeff: Yeah, I definitely see I definitely see a big movement to the edge and it is it’s these latency sensitive workloads that tend to drive you know, it’s why going back 15, 20 years, you know, it’s why you started to see CDNs built up for caching some of these videos out at the out at the edge because you wanted low latency and you also wanted to minimize some of the data as well.
So, things that are [23:00] lots of data and big. Yeah. and need to be low latency, those are really well suited to be sitting out at the edge. Now, what limits it sometimes is when the edge doesn’t have enough performance, then you make compromises and you move things further away because the source of the large processing is, you know, is somewhere else.
And so, I guess that’s where we sort of have two competing forces. Workloads that are getting bigger, that are more and more latency sensitive, they’re going to want to sit at the edge. And then there’s Kind of the role we play on the technology side, which is figure out how to deliver as much compute out to the edge at as low as, you know, the lowest power possible so that the intersection point of those things is as big as possible so that everything that wants those characteristics of the edge can sit there and doesn’t need to go somewhere else for processing.
And again, this same type of thing happened over the last, the last 20 years or so. And these workloads find their natural place. I think we, we had [24:00] there, there were big arguments 20 years ago, 15 years ago about the cloud and where things are going to sit between public cloud and private cloud and edge and how we’re going to create a taxonomy around this and, and what it was all going to look like.
And at the end of the day, the, the workload needs and the economics of it kind of end up settling it. And there will be a large amount of workloads that if we enable the right technologies, we’ll sit at the edge and we’ll want to be close to the user. And I guess that’s, that’s our role is to make sure that we you know, we make that feasible and economical.
And so, I, I, I really just, AI is just perfect example of a workload. That, that wants to sit at the edge wherever possible, has all the characteristics, needs to be low latency, lots and lots of data. Privacy can be an issue. There can be locality issues as well. I mean, it’s, there will be places where you’ll want to run different models, depending on what country you’re in or [25:00] what geo you’re in, right?
There could be language dependent. It could be policy dependent. And so, there’ll be a lot of reasons why inferencing is going to scale. Everywhere it’s going to be the biggest scale out workload that we’ve ever seen. And that’s one thing where I think as we think about AI, I think this competition has been good and how we talk about inference.
What gets lost a lot of times in the bigger picture is all AI gets thrown in together and training and inference get kind of thrown together. And the key here is that. Inference and training look incredibly different. So, a lot of things I’m saying here about AI inference, maybe aren’t true for AI training.
But, but we have to look at them, even though. From a workflow perspective, you train, then you infer from a compute perspective, the requirements are very different. And from a deployment perspective, the deployment requirements are very, very specific and they’re going to want different solutions.
Brian: Speaking of the Eds, Jeff, Ampere has a very interesting use case with Spacetech, which is a Chinese technology company that’s part of a larger real [26:00] estate enterprise. Now, we’ve covered this in a separate podcast and a case study with Spacetech CEO Sean Ding. And I encourage listeners to go listen to that story because it’s amazing.
Jeff, tell us about it from your perspective.
Jeff: We’ve been working across a pretty wide range of use cases for a while now. Everything from a cloud out to edge. And the kind of connect the tissue is that there’s a lot of analytics and AI that occurs in all those places. And so having a really high-performance solution that’s also really power efficient matters across that whole spectrum, but for different reasons.
And the case of space tech, you know, they provide a property services for a really large amount of real estate in China. And the challenge that they were facing is that there’s just more and more smart devices that are collecting data. That’s just generating a ton of traffic, but it also opens up a lot of service opportunities.
Things like facial and vehicle identification, [27:00] security services, managing certain assets on the properties like the Lifts. So, with all that dynamic data coming in, and all these opportunities to use that data in a smarter way, they needed a really high-performance edge AI server. To provide services, you know, along those lines.
And so that, that’s sort of where this originated was that they were using an existing x86 solution, but they needed something that was higher performance, given the change in data traffic and the change in opportunities, the growth and opportunities but they needed something that was also really power efficient at the same time.
And that’s where Ampere came into the picture.
Brian: Can you share any of the, the data that has been captured so far as, as these guys have implemented Ampere solutions?
Jeff: Yeah, yeah, I definitely can. You know, when you look at the workload, there’s obviously a lot of elements of the workload, but probably the two most critical pieces of it are video decode, because that’s often the data source that they’re using, [28:00] and then the other key element of performance is, is AI inference to actually generate the results that you’re going to take some action on and their previous solution was an x86 based solution.
And it had a discrete GPU in it. And so that’s their, that’s their baseline. And so, the key was to deliver much more performance for video decode and for AI inference than that solution but to still do so in a really power efficient way. When you look at those two elements, the video decode.
Our processor in this solution was able to decode 126p 25 frame per second video streams in parallel. And so that far exceeded what they were capable of doing with the existing x86 solution. Where it kind of comes full circle then is then taking that data and then running the, the inference model.
So, running double digit number of inference models in parallel. The solution that we provided is 2. 6 times faster [29:00] than the x86 solution. So, it delivered, yeah, a pretty big gain in, in performance. So that kind of made it a, that made it a no brainer to go ahead and, and utilize this, this solution, you know, a step function increase.
And what they were able to provide in terms of services to the properties.
Brian: In terms of that. x86 migration in this case, are you seeing that in other customer engagements as well in other applications?
Jeff: We are, we are, yeah, I, I think that you know, when you look at the market, there’s some small portion of the market where it’s Arm native applications in code, maybe it’s Android applications, maybe it’s something in the automotive space where for many, many years, the code has always been Arm based and that, that’s a no brainer to run that stuff on a on an Arm based processor like it.
Like Ampere processors, but there’s a really large part of the market that has traditionally been six code. And the, the issues [30:00] that that market’s facing is that running that x86 code on x86 CPUs, isn’t giving them the same gains that they are used to expecting over the last 10, 15, 20 years, obviously the Intel processors aren’t as competitive on a gen-on-gen basis as they once were.
And all of those processors are, are seeing large increases in power. So, it’s starting to make it difficult to utilize x86 processors for a lot of power efficient use cases. So, what I’m really seeing now is it’s not that there’s an arm market and there’s an x86 market. There’s a market that needs high performance, power efficient processors.
And that market is now moving their code from being x86 based to being arm based. And the good thing about this is that the places where that’s most common, the workloads where it’s most common, you know, these are a lot of cloud native workloads. These are a lot of workloads that use open-source code, and so it’s people running it’s people running Pytorch, it’s people running [31:00] MongoDB.
It’s people running NGINX. All of that code has already been ported over to, to Arm. It has been for a long time. And so, it’s not a lot of extra work for somebody to utilize the Arm base code versus the x86 code. It’s just a one-time switch. And so increasingly I’m seeing a lot of those traditional cloud and edge workloads moving off of x86.
over to Arm and it’s running Arm code natively on Arm based processors. And so, it’s really a seamless experience for the for the end user. Let’s come back to the
Brian: space tech case study for a second. So, this is an edge use case. And when you talk about modern workloads, AI workloads, it’s usually a GPU conversation.
But you guys are obviously CPU forward. You need GPU power and performance and presence in the data [32:00] center, especially around training. But as you move out to the edge, talk to us about that landscape. Yeah. GPU versus CPU.
Jeff: I would even cut it one step further. You know, I think it’s, it’s general-purpose CPU on one end.
It’s GPU, maybe on the other end, but just, just through a traditional lens. And then there’s domain specific accelerators that probably sit somewhere in the middle. And now today, certainly AI training and data centers is the domain of GPUs. There’s a decade plus of legacy there. These are. Big workloads that run for days, weeks, months, and big clusters.
And so, they look a little bit more like the GPGPU workloads of the past in supercomputing. But training workloads are things that can happen in one place. They don’t need to happen in a thousand different places around the world. Because you train the model once, and then you deploy the model. When you look at deploying that model or running these workloads, now it’s a totally different problem state.
It’s not running one workload for a really long period of time in [33:00] one place. It’s now running one workload that might run for, you know, nanoseconds to milliseconds. So, it needs really low latency. And then running that workload millions or billions of times in a short period of time. And those workloads have to sit really close to the end users that are getting the results because latency is really, really important.
So now the GPU solution that sits in that big hyperscale data center training the model, that doesn’t work in those places. It doesn’t work for a number of reasons. It doesn’t work because it could be trickier to deploy. It’s just a more complicated system. And that’s not what you need out of the edge.
You need something that’s much more compact and simple. It also tends to have a lot of thermal constraints, so it can be hard to cool some of those GPUs. They tend to consume more power and also, they can be significantly more expensive. When you’re looking for a big scale out solution that you can deploy anywhere.
And so, what somebody is looking for is really power efficient solution that’s flexible, that can handle a lot of different workloads coming [34:00] at it. It’s not just running one workload for a long period of time. Keep in mind, these edge servers or edge devices aren’t also just running AI imprints all day long.
You know, they’re also running all the other workloads that that need to be provided. In conjunction with instance, maybe a bunch of databases, a web server. There’s a bunch of caching pieces in there. I mentioned the video decode as well. So there needs to be a very flexible solution. And this is where CPUs are really good at this.
CPUs are general purpose. They can run any workload, which means that whatever you throw at it, they’re going to be able to handle. And so even when you don’t know what the demand is from second to second, a CPU does a really good job of handling. CPUs also are good in this use case because it’s a lot of data traffic.
And minimizing the number of hops that that data is making and keeping it in the, in the CPU can be the lowest latency way to deliver these results. So, you know, while a GPU can get the job done, it tends to do so a little less efficiently, more expensively and a little less [35:00] flexibly in these types of, of environments.
Now, I did mention there’s a piece in the middle where there is a need at times for different domain specific accelerators, but that doesn’t necessarily mean a GPU. That may mean a piece of AI acceleration hardware that’s really, really good at large LLMs, or it could be a piece of acceleration hardware that’s really good at computer vision, or like we see in NPUs and other types of DPU devices.
It could be that is really good at network. And so, I, I think that what we really see is you want really general-purpose performance matched up with a very, very domain specific acceleration. And that mix and match approach is a better solution. When
Brian: you sat down and started to hash out how this would look with the SpaceX folks, how much were they interested in the power efficiency story that, that you bring and how much were they interested in the, the code base, the.
Ecosystem [36:00] around which they could create their solution.
Jeff: Yeah. I mean, I think if you look at the requirements they had, I mean, the number one requirement was performance with the number one constraint. Being power. And so, you know, really their goal was delivering multiple X more performance, but still sit in the same power envelope.
So that was the number one consideration. And then I think similar with many other people, yeah, I think that the code base. And the overall ecosystem, you know, that’s table stakes. And so, once we were able to prove that this code ran just as easily on these devices with only minor changes, by moving to an Arm based code base and using the right SDKs then that became an easy part of the story.
But at the end of the day, you know, the reason why we’re seeing this big migration away from x86 is because there’s a need for more and more performance. environments that are constrained and x86 just doesn’t get the job done. And when I say constrained [37:00] environments, the obvious one is an edge where you clearly only have a limited amount of power, but frankly, hyperscale data centers are constrained today too.
It’s just a different order of magnitude.
Brian: We’re bumping up on time. We talked about workloads at the edge. We talked about workloads everywhere. Last question for you. What is your personal favorite AI application today?
Jeff: Man, my personal favorite. Well, actually I have young kids, so I would say that the two things they’re most entertained by right now.
So, one is one is using chat GPT to create stories. So, they, they love that one. They love feeding in goofy stuff and seeing what ridiculous, you know, stuff comes back out again. So that, that’s one that my, my kids really like the other one is stable diffusion. So, with some of the image generation models, I like this one for two reasons.
Also, one, it’s never been easier to create, you know, what is essentially you know, stock images. I mean, anything you come up with for a blog or [38:00] something else within seconds, you’ve got an image that kind of matches what you were thinking. But this is another one coming, you know, coming back to my kids.
They also love. Sending in crazy prompts and seeing what crazy image comes back again. I love the, yeah, I think it’s the creativity and the way that the LMS of today are able to kind of take the, the craziest things you come up with and then, you know, turn them into, whether it’s writing images, video instantly in ways that we never could have before.
It kind of always had to sit in your imagination and, and now they can kind of bring some of that stuff out and help you share it with other people.
Brian: Amazing times. We’re fortunate to live in them, aren’t we? It is. Well, Jeff Wittich, thank you so much for your time. Awesome conversation. And we look forward to having you on again in the future.
All right. Perfect. Well, thanks, Brian. I really enjoyed [39:00] it.