Tech Unheard Episode 5: Alex Wang
Podcast episode also available on the following channels:
Summary
In the fifth episode of Tech Unheard, Scale AI CEO Alex Wang joins Arm CEO Rene Haas to discuss the connections between humans, data and AI – as part of what Alex calls “humanity-first AI.”
Alex founded Scale AI in 2016 at the age of 19, with the company now working with Microsoft, Meta, OpenAI and more. He talks with Rene about why youth could be an advantage in the world of technology, while reflecting on his own upbringing among scientists near Los Alamos National Laboratory and learning how to manage stress through math competitions.
Tech Unheard
Learn more about the Tech Unheard Podcast series.
Speakers
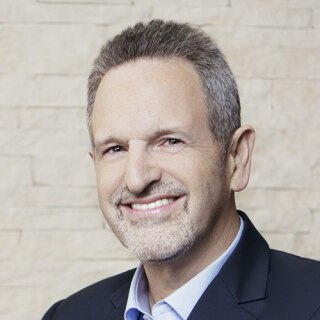
Rene Haas, CEO, Arm
Rene was appointed Chief Executive Officer and to the Arm Board in February 2022. Prior to being appointed CEO, Rene was President of Arm’s IP Products Group (IPG) from January 2017. Under his leadership, Rene transformed IPG to focus on key solutions for vertical markets with a more diversified product portfolio and increased investment in the Arm software ecosystem. Rene joined Arm in October 2013 as Vice President of Strategic Alliances and two years later was appointed to the Executive Committee and named Arm’s Chief Commercial Officer in charge of global sales and marketing.
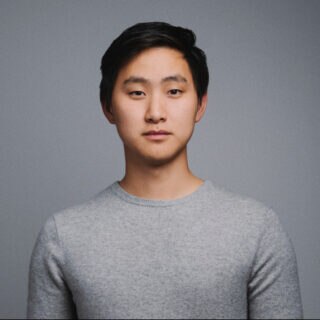
Alex Wang, CEO, Scale AI
Alex Wang is the founder and CEO of Scale AI, a company focused on “humanity-first” AI. Scale AI leads AI advancements across industries – whether in autonomous vehicles, defense applications, or large language models – ensuring these developments strengthen human sovereignty.
Alex founded Scale AI in 2016 as a 19-year-old MIT student with the vision of providing the critical data and infrastructure needed for complex AI projects. Under his leadership, Scale AI has grown to nearly a $14 billion valuation, serving hundreds of customers across industries ranging from finance to government agencies, and creating flexible, impactful AI work for hundreds of thousands of people worldwide.
Transcript
Rene 0:07
Welcome to Tech Unheard, a podcast takes you behind the scenes of the most exciting developments in technology. I’m Rene Haas, your host and CEO of Arm today, I’m joined by Alex Wang, CEO of Scale. AI. Alex founded Scale AI in 2016 at the age of only 19. Now Scale AI works with companies like Microsoft, Meta, OpenAI and more, as the API of human intelligence. Alex has become a major voice of AI, both in Silicon Valley and in Washington DC. Alex, thanks so much for joining me.
Alex 0:41
Thanks for having me. Super excited.
Rene 0:43
Yeah, thank you. One of the things I like to start with is folks background, and your background and my background are, are different, but not wildly different. I have immigrant parents as well. My dad didn’t work at Los Alamos, but he worked at Xerox in their research labs, and he came over when they were hiring a lot of Cold War scientists from the 1930s to come over and do work in the 1960s in the United States. So that’s what brought him over. And you grew up in Los Alamos, which is a pretty, pretty cool and unique place to grow up.
Alex 1:13
Yeah. So I was born in Los Alamos, New Mexico. My parents were part of, I think, many waves of scientists who moved to the United States in the 80s. They did their PhDs here, and then they, you know, for both of them, basically their first job in the US was at Los Alamos National Lab. And I think my mom, particularly has only worked for one employer ever, which is Los Alamos National Lab. So they’ve been very dedicated to the lab there. And Los Alamos is a very unique place to grow up. It’s the highest number of PhDs per capita, highest PhD per capita county in the United States. And it has this sort of continued air of, I would say, like, you know, high embrace of, sort of science technology, but also sort of, like, really embracing weirdness. And it was sort of a special place grow but it’s also quite small, you know, it’s about 10,000 people when I was growing up.
Rene 2:02
Did you go to a public high school?
Alex 2:04
Yes, there’s a great public school system in Los Alamos, as you might imagine, because most of the teachers are the partners of scientists. I mean, I think it probably has to be one of the better STEM educations in the United States, in public schools, just period.
Rene 2:19
I can imagine that they have off the chart math clubs and science clubs and computer clubs at early ages. But what did they have in terms of stuff that you get involved in early.
Alex 2:27
Yeah, so there were tons of clubs. I remember in like fourth grade, I was part of Rocket Club, where you assemble a model rocket and launch into space. Science fair was a really big deal. It was a very, very big and competitive science fair. And then when I was in middle school, I got very, very deep into math competitions. Particularly It was born out of a – it’s a story is kind of funny. There was, there was one math competition in particular called math counts, where if you place in the top four in the state, you would get an all expense paid trip to Disney World in Orlando, Florida. And at that point, this was in sixth grade, I don’t think I had, I traveled very little outside of New Mexico, and so this was sort of like an incredible incentive. And so I studied really, really hard. I managed to, like, barely get fourth place in the state. I got, I got, like, one point higher than fifth place. But then that was the start of me being sort of addicted to math competitions.
Rene 3:25
What was the competition? I mean, what were the problems you were trying to solve? Fifth grade? You said, sixth grade?
Alex 3:29
Sixth grade. Yeah, sixth grade. And the problems were, you know, it’s funny, because a lot of these problems now have made their way to AI evaluations and large language model evaluations, but they’re, they’re sort of brain teaser-y math questions. This competition was for middle schoolers. It was all about speed. And then there’s another competition I started doing that was much more about, sort of, like, you know, much longer form reasoning. So there’s, there’s a competition where you have six problems in nine hours. That problem set, by the way, those problems LLMs can’t get solved reliably, so, so the those problems are sort of hard enough.
Rene 4:04
I imagine for a sixth grader doing that kind of set, the stress level. I mean, what were you stressed out doing that? Or are you at that age kind of not, stress is not what you’re thinking about?
Alex 4:13
I think, yes, stress was, I think, because of competitions of various forms, whether it was I did math competitions and I did violin competitions and all sorts of things, I think I learned to manage stress pretty well from an early age. But, I mean, I remember there was one math competition where I accidentally I had coffee for the first time ever right before the competition. And that was like one of I remember it still is probably one of the most exhilarating experiences of my life. It was really, it was really quite, yeah.
Rene 4:43
I guess the competition didn’t do random drug testing for caffeine stimulants.
Alex 4:47
Yeah, exactly.
Rene 4:49
So was it that that kind of got you on the path to go do math and science in university?
Alex 4:54
Yeah. So, I mean, both my parents are physicists. And they work, both work on super secret stuff at Los Alamos National Lab. So I would say that the path to do STEM was kind of pre-ordained in many ways. And, you know, both my brothers, I have two older brothers, they both have PhDs in STEM fields, like it was obvious that I was going to do STEM, the deviation came from my parents are both physicists. They obviously would love if their if their children also did physics. I was very interested in physics, but I ended up going and doing computer science and AI. I think, when you grow up in the birthplace of the atomic bomb, and where all this incredible science has been done, and you read about it, and there was sort of this, like, you know, there’s this, like feeling in the air of incredible discovery, the closest place where that felt replicated in the modern day really was, was AI? You know that, AI was the field where it was like, Wow, there’s so much opportunity for discovery. There’s so many unknown, unknowns, and there’s so much progress.
Rene 5:50
Yeah, I want to come back to that, because I know we you chatted once publicly about Los Alamos and your background, atomic bomb. But when, when you were there, living there, did you have an overhang of feeling of significant scientific discovery was made there, and or was there a feeling that the ultimate weapon was also created there? I’m just curious, in terms of what the overall feeling was from folks who grew up there.
Alex 6:14
Yeah, it’s interesting. So there’s a museum in town called Bradbury Science Museum. It’s a small museum. You know, there’s like, a life size replica of fat man and another one of little boy. So there’s like, you can see what the bombs looked like. There’s a whole section dedicated to sort of, like the aftermath of the bomb. And what were the results in the war? What were the results that after the war? There was this big theater, and you would watch these movies, which were like pretty grim movies, you know, on the one hand, it would sort of glorify, certainly the the discovery. But you would, you know, 60% of the movies would be about, like the bomb actually going off, and like the damage it caused, and, you know, all this stuff. So I would say there was certainly a sense of responsibility, real responsibility given by sort of like the bomb and the implications of the bomb, but at the same time, there was also, like a real reverence to the the scientific discovery, as well as the sort of like convergence of scientific minds, so, you know, being able to coalesce, you know, Oppenheimer and Fermi and, you know, all the other incredible scientists who coalesced around the effort that was, I think, one of another sort of magical element.
Rene 7:24
So I’m very curious. So you went to MIT, and then very quickly decided you wanted to go off and do your own thing, which I always admire the courage of bright entrepreneurs like yourself who make that call. Tell us about how that all took place.
Alex 7:38
It actually starts a little bit before then. So through, through all these math competitions that I did, there’s a strong sort of, let’s call it like a mentorship community among among people who do math and science competitions at the national level. There were sort of a few of them who organized this, this summer camp called Spark is like the Summer Program for Applied Rationality. And then I don’t remember what the C was, maybe, and Creativity or something. So it was a summer camp where they coalesced a lot of very bright teenagers and a lot of people who had done these math competitions. And the people running, it happened to be some of the very early luminaries in AI. So one of the people running it was this guy, Paul Cristiano, who was, I think, one of the leading thinkers of AI safety, became like was an early member of OpenAI, invented RLHF, now runs the research at the US AI Safety Institute. The other person was a now professor at Berkeley, Jacob Steinhardt. Some of the speakers they brought in were like they had Greg Brockman, while he was still at stripe, coming to a speech. And this was in
Rene 8:44
Was it like, 2015, 2014?
Alex 8:46
Yeah, exactly. It was, 2014 was the first year I went. It was held in Berkeley, 2014 and 2015 I went both years. But I think the first time we went people, sort of like people went around and talked about what they’re excited about, 2014, remind you. And one person was like, I’m really excited about deep learning. And that word was meaningless to most people, it was like, What is deep learning? What even is that? It’s like, oh, you take you take neural networks, and you scale them up and you make them deeper and deeper, and all that sounded just like ridiculous. It was like. You’re doing what? You’re – what’s a neural network, like all this stuff. But I was very lucky to have gotten this, like, early glimpse into into the potential of AI, and this was at a point where, like, the, you know, the neural networks were not capable of much more than just, you know, detecting objects and imagery, or detecting of YouTube videos had cats in them. That was kind of the, I would say the state of the art at that point. But I was exposed to it so early. And these were a lot of people who were, you know, I saw a lot of people who were working on it at Google and and I was even exposed to a lot of the AI safety arguments, like, quite early on, yep. And then I went to MIT. So that was, that was in high school. I went to the summer camps, right? And then I went to MIT for a year. I really wanted to study this AI thing. So I took as many, like, all the courses that I could at MIT.
Rene 10:00
For a freshman what was there?
Alex 10:02
So I lucked out my freshman advisor, who is the person overseeing my curriculum and stuff, she actually was the Professor of the graduate machine learning course. And so I sort of told her, I was like, Oh, I’m, you know, I would love to take your class, you know, I would really love to be able to learn from you. And she was sort of the skeptical, as, you know, as it were, but the first semester of MIT has passed no record. And so she’s like, sure, you can try to take it, but like, you know, we’ll have to monitor your progress. And then, quite luckily, I think it was like, truly luck, the first exam I did extremely well. I think I, I think she told me I got, like, one of the top few scores in the in the whole class, in the class, a few 100 people.
Rene 10:44
How did you, by the way, how did you convince her? I’m very impressed. Now, in terms of, as a freshman, convincing your advisor, let me go off and take this graduate deep learning class.
Alex 10:51
It was, you know, as you as you find out in in general, in life, a lot of things, if you have a common connection, it becomes much easier. So I did this internship, and I worked at Quora, the question and answer website. And there was a co worker of mine who had done research, and the Professor was Leslie Kaelbling, in Professor Kaelbling’s lab, you know, years and years ago. And so it was sort of –
Rene 11:12
Got it, got it, yeah, you were already on to the magic of connections.
Alex 11:15
Yeah, the magic of connections. So I took that course. I took all these courses when I was at MIT, was the year when Deep Mind came out with AlphaGo, when Google released TensorFlow. And so deep learning, this thing that I had like heard about years of years before, was becoming more mainstream, and so I wanted to try to apply it. So I tried to leverage it to build a camera inside my refrigerator that would tell me when my roommates were stealing my food. And and I worked on and I worked on, and I was like, wow, this is, like, this is, like, this magical thing. You just put in a data set, and then you press go, and, like, then you get a model spit out. And it’s like, if your data sets really good, the model is really good. And so then I was like, Oh, wait, that means the data set is really, really important. The data set is kind of doing the programming, so to speak. And that was really the origin behind Scale, this sort of like realization that data was going to be this, like critical, critical component of all future progress in AI.
Rene 12:13
Yeah. I mean, to some extent, Alex, you got into this both because of your amazing capability, but you got started at a time where everything kind of came together because, you know, the ideas of neural nets and deep learning were sort of academic papers, but the technology had never really kind of come together to allow it to all coalesce. If you go back to that moment of AlexNet, and I know publicly you state you’re not chips guy, but you’re a bright, bright guy. What was the big bang moment you think that’s enabled all of this to come together? Was it the acknowledgement of the data set? Was it access to compute? Was it a chip that now is actually fairly good at programming parallel models for neural nets? What do you think kind of brought it all together in that time?
Alex 12:56
I think it was really all the above, by the way, AlexNet – well, yeah, at the time, there were a few things that happened at once. One is Fei Fei Li in her lab, created ImageNet, which was at that time, you know, this like massive, massive label data set. There was never, there was never any data set that large that anybody was doing serious machine learning on. And in fact, it was quite visionary from her, because it was, it was a size at which I think most people in the field would have assumed, like, hey, there’s no point in even having a data set that big, because, like, the models are just not going to be able to, you know, you’re not going to have enough computational power to learn from all of that. So the first was like, there’s this massive data set. Then there was AlexNet, which, by the way, AlexNet was trained on CPUs, not GPUs originally. It was very brute force-y almost. And then AlexNet was this, like, you know, it was this crazy thing. It was, like, this incredibly, this, like, pretty crazy idea of just leveraging all that data, training this big neural network. It was not very intentional, which I think was very frustrating, like the way that, the way that AI has gone as a field, is very academically unsatisfying, because it’s so powerful, but it’s not very, it’s not very clever, it’s not very intentional from an academic perspective. And so you had AlexNet. And then you just had these, like early visionaries, many of whom sort of went on and really shaped the whole field, who realized that, like, we really are just going to be able to to scale this up, right? Like you have to optimize the compute, you can scale up the networks, you can scale the data, like you do all these things, and you’re going to get incredible outcomes. And that’s a really unsatisfying answer from a scientific perspective, because, you know, the the answer is kind of like, oh, yeah, you just do what we did before, and you you just scale it up, right? There’s no other tricks. But, you know, there were obviously some tricks along the way, but for the most part, like, it was this confluence of, you know, these massive datacenters being built up for for web 2.0 reasons, basically, for, like, the sort of like social and and, and search era. And then so you had access to all this compute. And then you, because the internet, you started getting the ability to produce very, very large scale data sets that you weren’t able to produce before. And then you had these, like brilliant scientists who were like, working at this intersection. And these sort of three things came together.
Rene 15:14
Well, you, I mean, I want you to tell our folks what Scale AI does, but you very early. Not only you got into creating something new, but you very quickly got into the, understand the answer is that it’s actually all about the data and really, really linking that fast, which, again, is just just brilliance. Tell our listeners, how did you immediately kind of understand that? You know what? This is a data problem at the end of the day, more than anything else.
Alex 15:40
Well, first Scale, we really think about ourselves as the humanity first AI company, and I’ll get to how that connects with data. But we see ourselves as the only company in this space that will be focused on keeping humans at the center of AI. How this connects to data is really like, you know, if you really boil it down, all the data that fuels these models, these AI models, are generally produced by humans, because, you know, what we’re trying to do is produce intelligence. And the best form of intelligence that exists in the world right now is human intelligence. So our business has, sort of has two sides, infrastructure and applications and and at the heart of, you know, all these problems in AI, we really see it really boils down to data problems. So what we do in our infrastructure side of our business is we generate massive scale data sets. We’re probably the one of the largest proprietary data sources, probably the largest proprietary data source for most of the model providers, I mean, we produce these large scale data sets to fuel AI development. And this is we’ve done this through many phases of AI.
Rene 16:42
And quick question now, when you say produce this is, is this synthetic data and or mining enterprise data that is just scattered, not able to be easily accessed, etc, etc,
Alex 16:50
Yeah, it’s a mix. First, we have a network of human experts, so the large network of human experts who actually help us produce very, very high quality data. Then we use, we have, we called hybrid data, this combination of, you know, the insight those experts can provide, as well as synthetic methods to produce, sort of, to amplify, sort of, their signal and produce a lot more data. And then we have, we work directly with governments and enterprises to help them harness their proprietary data for their specific applications, of which there’s immense amounts. I mean, like one stat which is just staggering, is the GPT4 training set, I think is about a petabyte, maybe half a petabyte. And then the, JP Morgan’s entire, sort of like corporate data set across all of its data is in the hundreds of petabytes.
Rene 17:38
That’s insane.
Alex 17:39
Yeah, the amount of data, truly, the amount of data that’s out there that’s being unused for AI training today is really astronomical.
Rene 17:46
Quick question on that, what percentage of that 160 petabytes could ever actually be? Is it an unsolved problem? Can you, could you ever get to all that data in some way, shape or form?
Alex 17:57
It’s a good question. There’s, well, yeah, there’s immense, immense data sets. You know, we don’t know exactly how we use all of it. And the truth is, like, a lot of it is probably not actually that useful and valuable, but the pieces of it that are really, really valuable, you know, we have methods of using a lot of it, but not all of it. So the models now are very multi-modal, so you can probably use, you know, all the audio data, you can probably use all the video data, you can probably use all the text data, you can probably use a lot of the structured data. But, you know, the exact way that you do that is sort of like we’re still figuring out the exact method, but ingesting all this data and leveraging the truly networked way in which all of all enterprise data or government data is actually sits, is sort of not yet fully known.
Rene 18:38
And is there a ceiling? You’ve got human agents who are gathering intelligence. Again, the stuff you guys are doing is so unique. And I’ve heard you talk about the data wall, and the data wall is kind of the end quote, public data, but using the methods that you guys apply, in other words, the humanity component, and adding intelligence on top of creativity. Is there no limit? Is there just, you look at it and say, there’s just no ceiling to the amount of data that could be generated, or at some point, does it asymptote in terms of you’ve got what’s called data, but not information.
Alex 19:08
I don’t see a ceiling. So the data wall, as it’s often talked about in the industry, really has to. With the fact that, you know, we got very lucky, which is that humans have been producing one of the most valuable data sets over the past few decades, randomly, very naturally, which is called the Internet. And so a lot of lot of the data that these models are trained on is the public Internet. But that internet, you know, it only grows every year at a certain rate, which is much, much slower than, unfortunately, we need to grow for these models. And so the data wall really is that like, Hey, we’ve run out of the internet, basically. And how are we going to produce new data? How are we gonna have data abundance that gets us beyond this sort of like this, like scaling limit. And so we don’t see a limit to new data that we can produce or leveraging, you know, proprietary data from enterprises or governments. Like, we don’t see any near term limit from these data sources. And I think long term in the industry like, you know, there the amount of hunger of AI models for data is is fundamentally insatiable, you know. And, and we’ve seen this like time and time again through every phase like, you know, we started working in autonomous vehicles. That was the, you know, when we when scale got started in 2016 that was the hotness. And that was the that was the hot industry. And even in autonomous vehicles, a lot of the conversation was like, you know, and conversation even now is like, you know, is Tesla’s approach better where they just have millions of vehicles on the road, but they don’t have LiDAR, or is the Waymo approach better where they have LiDAR and they have all these advanced sensors, but, you know, they may be more limited from the number of vehicles. And the answer is, like, both just need so much data. You know, the Tesla vehicles, their models, are still very data hungry, the Waymo vehicles, like every new city, requires, like, you know, whole new data set to be able to train the model effectively. So, the answer is, like, they’re both ridiculously data hungry. And then we’ve seen that, you know, go from that era of early robotics and self driving to LLMs and reasoning to now in one of the newest areas is robotics, back again, you know, humanoid robotics, or other forms of robotics, and those are going to need incredible new sources of data. It’s one of these, like, incredibly fascinating industries. But the, you know, there’s a few things that are just like will always be invariant. You know, models will always want more data, and models will always want more computers and those, that will never change.
Rene 21:40
It feels that way to me. I mean, I’ve been involved in so many trends over the years, whether PC, mobile phones, internet, and everyone says at some point it asymptotes, and you’re on the next thing. But artificial intelligence, which is the quest for information humanity, for as long as humanity has existed, has had an insatiable quest and appetite for information, and that is why, to some extent, I think this is a little bit different. This has been fascinating. I would go on for another hour on this, but I want to ask you just a few other questions in the last few minutes we have left. You’re a really young guy leading a really big company, you know, I’m a CEO running a decent sized company, and I’ve had lots and lots of years of experience. I’ve had lots and lots of bosses, and it took me a long time to get where I’m at. So I look at someone like you, and they say, You’re doing such an amazing job leading a company at such a young age. Who are your mentors, who are your coaches? How did you learn to become I mean, first off, you’re a brilliant guy in terms of the technology piece, but you’re also leading a company and a very big company and a very valuable company. How do you do it? Who’s taught you, who’s taught you to be such a great leader?
Alex 22:49
Yeah, one thing I think that I think most young founders learn, and I certainly learned this is that being young is both an incredible advantage, because you’re naive and you don’t have all the battle scars you’re going to push for the things that fundamentally you know are right. You won’t have like, all these like guardrails built into the way that you operate. It also can be very tough because you have to very, very quickly learn how to be a good people leader and be a great leader of like, a large organization, which is ridiculously hard, is very, very difficult. And so for me, I’ve been very lucky because I’ve had some great mentors through the year. So so one of them is Jeff Wilkie, who ran Amazon consumer for many, many years, I think, literally, like over 1 million person organization, absolutely. Jeff’s amazing. And he’s an incredible, um, people leader. Um, some of our investors have been, has been really, uh, investors and board members have been really instrumental through the year. So Mike Volpe, Mike’s amazing too. Yeah, he’s seen, he’s seen a lot, and has been able to teach me. And then I think that I’ve honestly learned a lot from the leaders that have joined me in scale, in the journey, as well. So I think a lot of a lot of our executives, all of the leaders that we’ve we’ve brought in, have really taught me a lot as well. So I’ve been lucky to get a lot of. Help. But it is not easy. And my take is, you can, you can run an organization very effectively at smaller scale, but once you hit a certain level of scale, you know, you need totally new muscles.
Rene 24:24
And do you think you’re there yet?
Alex 24:25
I’m, I think I’m like training the new muscles and and then there will be a new another, another set of scale, and you’ll need totally new muscles. Like, it’s a it’s really unintuitive. I think, extremely unintuitive.
Rene 24:36
Any idols heroes when I, when I was getting into tech, I was, you know, he was about 10 years older than me, but Steve Jobs was always just somebody. When I was a high schooler and Apple was creating their first Apple two products, their first Apple Computer, I just looked at what jobs and was was doing and just they were, Jobs was always my hero. How about, how about for you?
Alex 24:53
Well, Steve Jobs is sort of obviously, really quite a quite amazing what he did at Apple is still sort of like singular in many ways. So I’m very inspired by Walter Isaacson’s biography of him. I was very inspired early on by Brad Stone’s books about Amazon, so everything store that was very meaningful. And so I think that we’re, we’re lucky to be able to, you can learn a lot about these companies and their leaders because of all the great writing that’s out there.
Rene 25:23
Absolutely and the Isaacson book, you know, having, having followed jobs for decades, was sort of the tell all, because they were just, he was the first one to ever write a biography about jobs, where jobs gave him access and actually spoke to him on the record. And it’s a great book. Our CFO is former Amazon and I spent a lot of years at at Nvidia under Jensen. So under Jensen, under Bezos, you know, we kind of have vicariously lived through a lot. So Alex, I could go on for hours with all the questions I want to ask you, but I want to thank you mostly for your time and congratulations on all the success that you’ve had there. And as you guys collect humanity and all the world’s data, we hope to help you at arm, because we have access to a lot of that data. So thank you again for spending the time with us.
Alex 26:06
Yeah, of course. Thanks so much for having me, and you’re a great interviewer. Renee, thank you.
Rene 26:16
Thanks for listening to this month’s episode of Tech Unheard. We’ll be back next month for another look behind the boardroom door. To be sure you don’t miss new episodes, follow Tech Unheard wherever you get your podcasts. Tech Unheard is a custom podcast series from Arm and National Public Media, and I’m Arm CEO, Rene Haas thanks for listening to Tech Unherd.
Credits 26:37
Arm Tech Unheard is a custom podcast series from Arm and National Public Media. Executive Producers Erica Osher and Shannon Berner. Project Manager Colin Hardin. Creative Lead Producer Isabelle Robertson. Editors Andrew Meriwether and Kelly Drake. Composer Aaron Levison. Arm production contributors include Ami Badani, Claudia, Brandon, Simon Jared, Jonathan Armstrong, Ben Webdell, Sofia McKenzie, Kristen Ray and Saumil Shah. Tech Unheard is hosted by Arm Chief Executive Officer Rene Haas.