Seven Hardware Advances We Need to Enable the AI Revolution
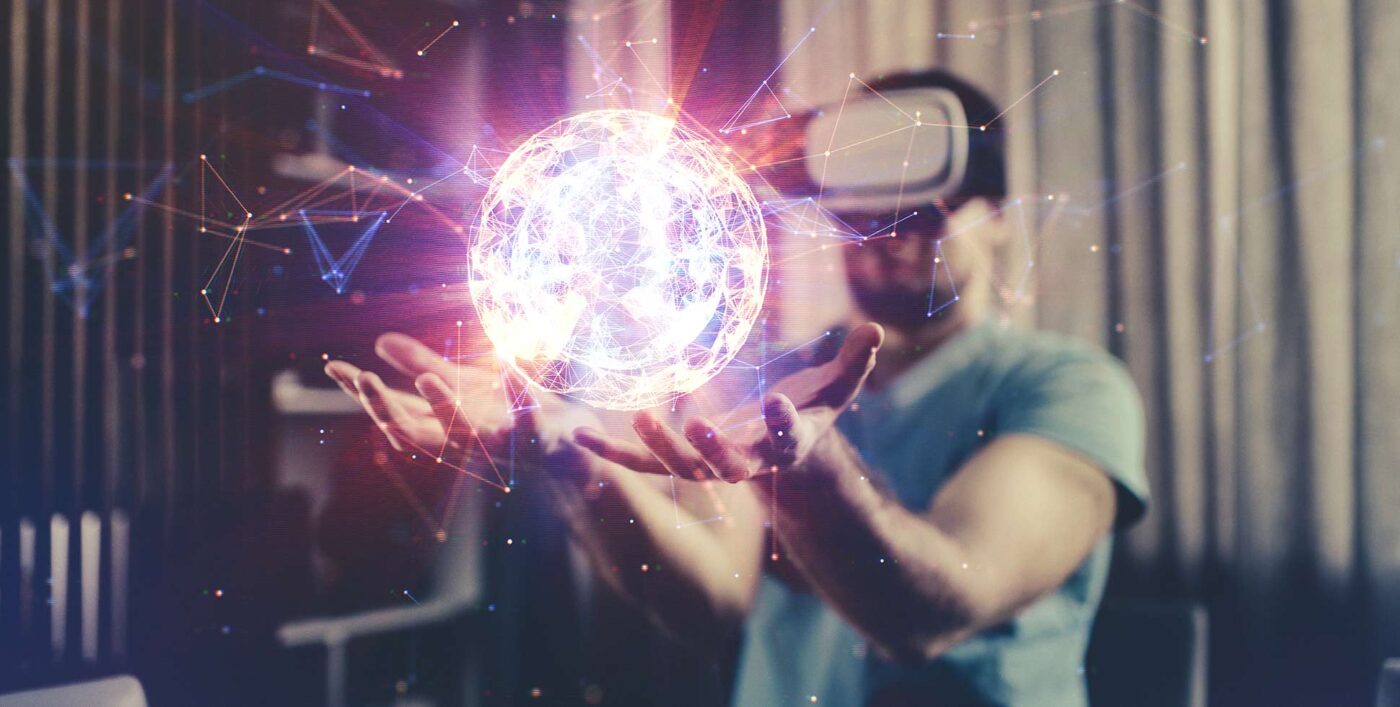
The potential, positive impact artificial intelligence (AI) will have on society at large is impossible to overestimate. Pervasive AI, however, remains a challenge. Training algorithms can take inordinate amounts of power, time, and computing capacity. Inference will also become more taxing with applications such as medical imaging and robotics. Applied Materials estimates that AI could consume up to 25 percent of global electricity (versus 5 percent now) unless we can achieve breakthroughs in processors, software, material science, system design, networking, and other areas.
There are two main directions for compute and AI technology development today: extreme-scale systems and edge/pervasive massively distributed systems. They both come with a mix of similar and diverging challenges.
From a hardware perspective, here are what I believe are the principal areas needing improvement.
1. Specialized Processing
Computing architectures hit an important turning point in 2006. Achieving performance gains through Mooreās Law and Dennard scaling became more expensive and problematic. At the same time, co-processors were making a comeback. NVIDIA released the G80, its first GPU targeted at servers that year. The first efforts to develop AI processors also started at the time.
Since then, GPUs have become pervasive in AI high-performance computing (HPC). Over 50 companies are developing AI processors, including Google, Qualcomm, Amazon, Facebook, Samsung, and many others. And Data Processing Units (DPUs) for network, storage, and security are becoming a permanent fixture in clouds and exascale computers.
The challenge over the next three-plus years will revolve around finding the magically delicious combination for different AI applications. Will cloud-based machine learning (ML) training be best served with wafer-scale processors or chiplets in Exascale computers? Or what level of training should take place on devices in a massively distributed system? We have a good portion of the core technology for both cloud and edge AI. What we will need is more AI dedicated architectures, together with intelligent ML-based dynamic system configuration and optimization.
2. Near Data Processing
Over 60 percent of the energy used by computers gets consumed in shuttling data between storage, memory and processing units. Reducing or even eliminating a large portion of this digital commute can significantly reduce power consumption and reduce latency. Processing-in-Memory, where tiny, dedicated processing unit is integrated into random access memory, will make sense in datacenters and exascale compute in generals.
At the edge, being able to process data in-sensor, or at least before it gets streamed or sent to the remote device could be a way to massively reduce the transit and storage of data. Only meaningful events or data would be transferred to a remote service and only when an intelligent engine at the edge would have said so.
Like specialized processing, this is a near-term innovation.
3. Non-CMOS Processors
As I wrote in my last article, low-cost, easily integrate-able processors made with flexible transistors and/or substrates will pave the way for reducing food waste, finding water leaks or encouraging recycling. Some of these tags will simply be smart sensors sending raw data, but increasingly they will leverage machine learning to reduce data traffic and elevate the āvalueā of their communications.
Arm Research, in conjunction with PragmatIC Semiconductor, last year showed off PlasticArm, an experimental penny-price printed neural network with sensors for these tasks. Processor designs, EDA tools, manufacturing equipment, and software will all need to be further developed and integrated into an end-to-end printed-electronics-asāa-service platform. Identifying a killer application will determine the next step and development speed for this domain.
4. Event-Based/Threshold Processing
Prophesee has developed an event-based image processor with pixels that operate independently of each other. Data gets updated only when changes occur, not on a synchronized cycle across the imager, similar to how the human eye functions. This massively reduces the amount of data captured, enabling speeds of up to 10,000 frame per second. Energy consumption, latency and computing overhead are all slashed while image resolution is enhanced.
Imagine taking an image of a downhill ski race: the body mechanics of an individual racer could be captured in minute detail by eliminating unnecessary updates of a static sky. Car crashes could be more accurately reconstructed.
Beyond computer vision, event-based sensory devices could be used to streamline vibration analysis, voice recognition and other compute in data-intensive applications. Imagine a smart tattoo that conveyed only meaningful events about your bio-signals to your smartwatch or health care provider after a threshold or chain of event is achieved. You would be able to monitor in real-time on a stream of data, with a tiny compute system, certain event characteristics of a system state, or of human emotion, or to predict divergence in certain cognitive diseases.
5. Neuromorphic Processors
It is possible to design artificial spiking neural networks or more generally, electronic components, in a manner that takes inspiration from the architecture of the human brain. Carver Mead first theorized about neuromorphic processors in the 80s. But still today, only a few experimental chips such as SpiNNaker 1 and SpiNNaker 2, a 10 million core processor platform optimized for the simulation of spiking neural networks, exist.
Neuromorphic computing seems very promising but continues to require breakthroughs in model training, ML dev-ops tools, and other technologies. We also need hardware that fits different use cases: wafer size chipset will not work for low-power oriented applications. Although neuromorphic research has mostly been targeted at exascale systems, it may make sense to concentrate as much energy on applications like ultra-low power keyword spotting, event detection for autonomous vehicles, or other data streaming processing use cases. Progress could come more quickly, and breakthrough concepts could be scaled up. The future killer application for Neuromorphic may not be in Exascale systems but more in low power oriented edge compute.
6. Extreme Ambient Cooling
Datacenters have been planted in abandoned mines, subterranean bomb shelters, and city harbors to reduce mechanical cooling loads. Liquid cooling also appears to be making a comeback.
Cryo computing if specifically designed to benefit from the physical phenomena that come at cryogenic temperatures could deliver significant benefits in performance per watt. What is key is to explore design optimizations from materials-to-devices-to-systems. An industry effort will be needed to bring the technology to life for large-scale applications in data centers and/or for Exascale computing systems, but the initial investigations look very promising and worth deeper exploration.
7. Zero Compute Architectures
if we look further at potential bio-inspired models, we could explore how to reproduce the way our long-term implicit memory allows us to efficiently accomplish known yet complex feats like driving a car in reverse or reading a book by merging step-by-step processes into a relatively automated procedure.
In a computing world, the system would be able to rely on learned or experiential functions, to shortcut compute-intensive tasks when they have already been performed once. At a high level, a zero compute system would include a mechanism that can recognize whether or not an application is new or learned, a process for executing learned tasks, and a library of learned functions for future replay. We could of course argue this is not truly zero compute but near-zero compute. Nonetheless, it could cut a tremendous number of calculations.
As with humans, weād have to be mindful of the trade-offs between performing tasks in a rote manner and critically examining every process. But assuming the balance works between a large number of known tasks, versus re-computing, we could imagine an Exascale intelligent system splitting the world of computing between known and unknown and distributing the answer to a massive amount of dumb systems.
This of course is just the start. As AI spreads, so will the need for more performance and efficiency at the hardware level.
The future of ML shifts to the edge
Learn how Arm partners are bringing AI and ML to the smallest of devices in this interactive report.
This story originally appeared on Semiconductor Engineering
Any re-use permitted for informational and non-commercial or personal use only.